So-called photonic neural network systems, which are quick and energy-efficient, are particularly beneficial for dealing with huge amounts of data.
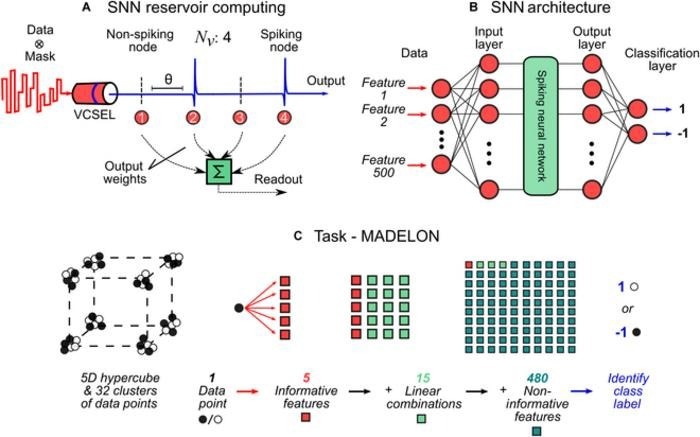
a) The operating principle of the photonic spiking neural network; b) The architecture of the photonic spiking neural network; c) The MADELON classification task. Image Credit: Dafydd Owen-Newns et al.
To progress photonic brain-like computing technologies, a research team at the University of Strathclyde integrated a spike-based neural network along with a semiconductor laser that displays spiking neuronal behaviors.
They demonstrated high-performance photonic spiking neural network operations along with lower training needs and initiated a novel training scheme for improved outcomes.
This study was reported in the journal Intelligent Computing, a Science Partner Journal on August 29th, 2023.
Neural networks that have gained inspiration from networks of biological neurons have revolutionized artificial intelligence by offering effective solutions to complicated tasks. To additionally unfasten their potential, scientists have been putting up ideas for other technologies into artificial neural networks and have also witnessed some success.
A successful example of the advantages of using light is that light-based or photonic neural network systems function with faster speed and improved energy efficiency. This makes them particularly helpful for handling huge amounts of data and hopeful for future artificial intelligence applications.
The spiking neural network suggested by the authors relies on a hardware-friendly photonic system comprising just one vertical-cavity surface-emitting laser, a commonly available device that is seen in mobile phones.
This proposal signifies an enhancement of a previous study conducted by the same group of authors. In their earlier work, they integrated reservoir computing, a potent technique for constructing photonic neural networks capable of addressing intricate tasks, with a neuromorphic spiking neuron developed using a laser of the same class.
In the current study, the authors accomplished a highly difficult classification task and employed an alternative training scheme to enhance the speed and efficiency of training while decreasing training needs.
The classification task handled by the researchers is a highly complicated one, a multivariate and nonlinear issue including around 500 features for every data point, and is dependent on the artificial dataset MADELON.
For the spiking neural network to be created, the authors made use of an experimental setup integrating the nonlinear spiking dynamics of the laser along with an architecture inspired by reservoir computing.
In this architecture, the input data has been time-multiplexed, that is, split into different time slots. Each slot represents a virtual neuron in the neural network.
The input data has been injected into and processed by the laser, and the output is taken as a binary node output, either spiking or non-spiking, based on whether the input data surpasses some threshold.
The authors illustrated the computational power of the photonic spiking neural network successfully with both a traditional least-squares regression training technique and their newly suggested “significance” training method.
The latter approach assigns binary weights to nodes depending on their overall utility and importance. Both techniques achieved remarkable classification accuracies exceeding 94%, outperforming the established benchmarks in a significantly reduced processing time.
Specifically, the new method attained accuracy rates of 94.4% and 95.7%, surpassing the results obtained through the traditional method. Notably, the spiking neural network trained using the new approach exhibited exceptional performance, even when trained on small datasets containing fewer than 10 data points.
The suggested photonic spiking neural network exceeds conventional digital semiconductor processing systems along with its low power consumption, ultrafast performance, and hardware-friendly implementation that makes use of just one laser to process all virtual nodes.
The authors believe that the study could create new chances for photonics-based processing systems that function completely on optical hardware, allowing them to handle highly complicated tasks with high accuracy, high speed, and energy-efficient operation.
Journal Reference
Owen-Newns, D., et al. (2023) Photonic Spiking Neural Networks with Highly Efficient Training Protocols for Ultrafast Neuromorphic Computing Systems. Intelligent Computing. doi.org/10.34133/icomputing.0031