A segmented primary mirror is crucial for detecting the phase error between segmented mirrors for extremely large astronomical telescopes. Due to the lengthy iterative procedure, traditional iterative algorithms have difficulty detecting co-phasing aberrations in real-time. Deep learning has demonstrated significant promise in wavefront sensing and is currently concentrating on identifying piston inaccuracy. The existing deep learning-based approaches focus on coarse phase sensing and only identify piston defects without considering tip/tilt errors, which is inconsistent with reality.
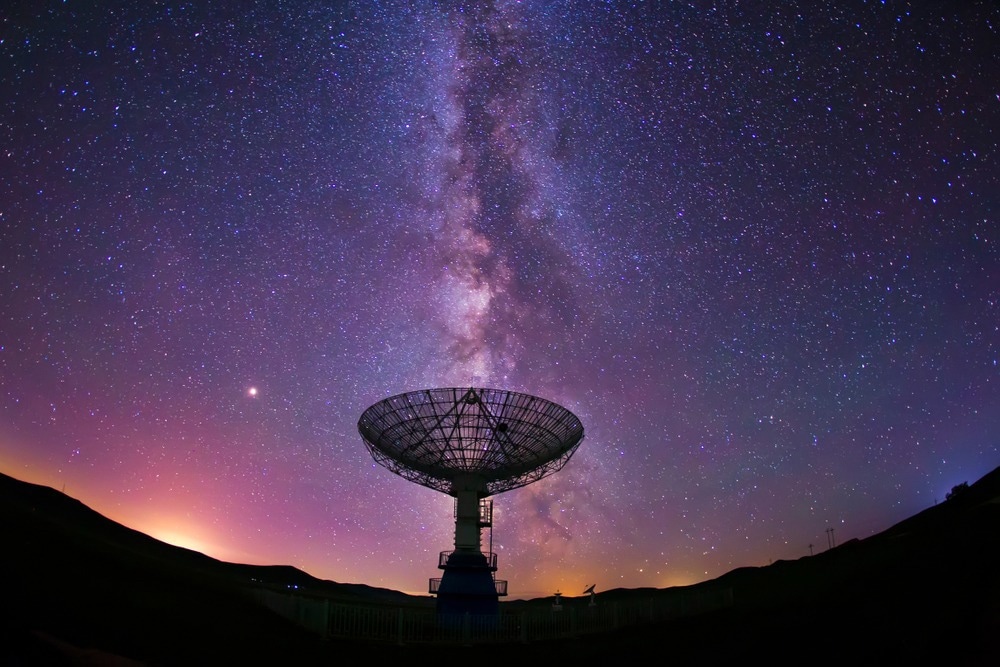
Study: Decoupled Object-Independent Image Features for Fine Phasing of Segmented Mirrors Using Deep Learning. Image Credit: zhengzaishuru/Shutterstock.com
Novel Decoupled Independent Image Feature
A recent study published in Remote Sensing demonstrates a novel decoupled independent feature image that can simultaneously sense the tilt/tilt and piston errors of all sub-mirrors. Decoupled independent feature image eliminates the data set requirement by designing a pupil mask and updating the OTF in the frequency domain. The phase error information is accurately recovered from the feature image using the Bi-GRU network.
Segmented Space Telescopes
Segmented space telescopes define the direction of development for aperture space telescopes. Primary mirrors with segments can solve various issues, including the production, testing, and launch of massive monolithic mirrors. The alignment of the system and the misalignment of the tip/tilt aberration and relative piston of each sub-mirror determine the imaging quality of a segmented telescope.
Limitations in Solving the Misalignment of Sub-Mirrors and Co-Phasing Problems
Misalignment of sub-mirrors can be solved with the help of sensors such as the Zernike phase contrast sensor, pyramid sensor, curvature sensor, Hartmann wavefront sensor, and Mach Zehnder interferometer sensor. Special sensors swiftly analyze the wavefront status. However, using sensors to predict co-phasing faults in practical applications is challenging and expensive.
The image-based wavefront sensing technique's phase diversity (PD) and phase retrieval (PR) components have steadily gained popularity. Only point target wavefront sensing is possible with PR. PD is appropriate for wavefront sensing of point targets and extended targets. An iterative approach uses an optical diffraction model to determine the co-phasing errors. The iterative process has the drawback of high computing and low robustness (stagnation problem), particularly when the object is unknown.
Long-term on-orbit working conditions are complex and subject to a wide range of micro-vibration disturbances, which can seriously impair imaging quality if point targets (fixed stars) with enough brightness are not present in the field of view. Therefore, detecting co-phasing problems in extended sources is crucial in real-time.
Potential of Deep Learning in Co-Phasing of Segmented Mirrors
Numerous deep learning applications have demonstrated potential in co-phasing of segmented mirrors. Deep learning approaches include Fourier ptychography, phase unwrapping, scattering medium imaging, and image restoration.
Deep learning has benefits over conventional PR or PD techniques, such as resilience (no local optimal problem) and a quick real-time analysis (no iterative process).
Deep learning techniques have no negative impact on co-phasing sensing. However, due to the coupling relationship, almost all co-phasing methods based on deep learning cannot concurrently detect tip/tilt and piston errors for all sub-mirrors in extended applications, which renders many methods invalid and significantly lowers detection accuracy.
Decoupled Object-Independent Feature Imaging for Co-Phasing of Segmented Mirrors
Wang et al. developed a decoupled object-independent feature imaging technique by constructing the pupil mask and updating the OTF in the frequency domain. This can concurrently detect the piston error and tip/tilt errors of all sub-mirrors. The data set no longer depends on the imaging object owing to the effective decoupling of piston error and tip/tilt errors provided by decoupled object-independent feature image.
The need for an additional optical diffractive component is avoided with this technique. The researchers also employ the Bi-GRU network to build a specific relationship between the extracted feature image and the phase aberrations to achieve the sub-aperture precise phasing of the extended scenes.
Research Findings
This study suggested a new decoupled object-independent feature image that effectively decouples piston and tip/tilt errors and eliminates the dependence of the data set on an imaging object. It can simultaneously obtain the phase information of all sub-mirrors. Limited hardware is needed.
It also eliminates the need for extra optical diffractive components in the conjugate plane of the segmented primary mirror. Extraction of new decoupled object-independent feature image predicts piston and tip/tilt defects efficiently and accurately.
The aberrated wavefront map is the only source of information for the new decoupled object-independent feature images. The aberrated wavefront map and feature images have a special mapping connection that allows segmented telescopes to achieve end-to-end co-phasing. The researchers compared the phase prediction accuracy of different new decoupled object-independent feature images for the fine phasing problem under identical circumstances. Both theory and simulation confirm the utmost precision of the new feature.
One network (Bi-GRU) was used to create a precise nonlinear mapping between the phase data and derived feature images. The solution suggested in this research does not require a deep and sophisticated network because the network requires little computer configuration. The fine phasing method based on deep learning can forecast the phase considerably more quickly and does not require an iterative procedure, which matches the real-time correction.
Reference
Wang, Y., Zhang, C., Guo, L., Xu, S., & Ju, G. (2022). Decoupled Object-Independent Image Features for Fine Phasing of Segmented Mirrors Using Deep Learning. Remote Sensing, 14(18), 4681. https://www.mdpi.com/2072-4292/14/18/4681
Disclaimer: The views expressed here are those of the author expressed in their private capacity and do not necessarily represent the views of AZoM.com Limited T/A AZoNetwork the owner and operator of this website. This disclaimer forms part of the Terms and conditions of use of this website.