Researchers have recently developed an advanced form of photonic spiking neural networks (SNNs), a significant step in neuromorphic computing. This new approach utilizes a multi-synaptic photonic SNN, combining modified remote supervised learning with delay-weight co-training for pattern classification.
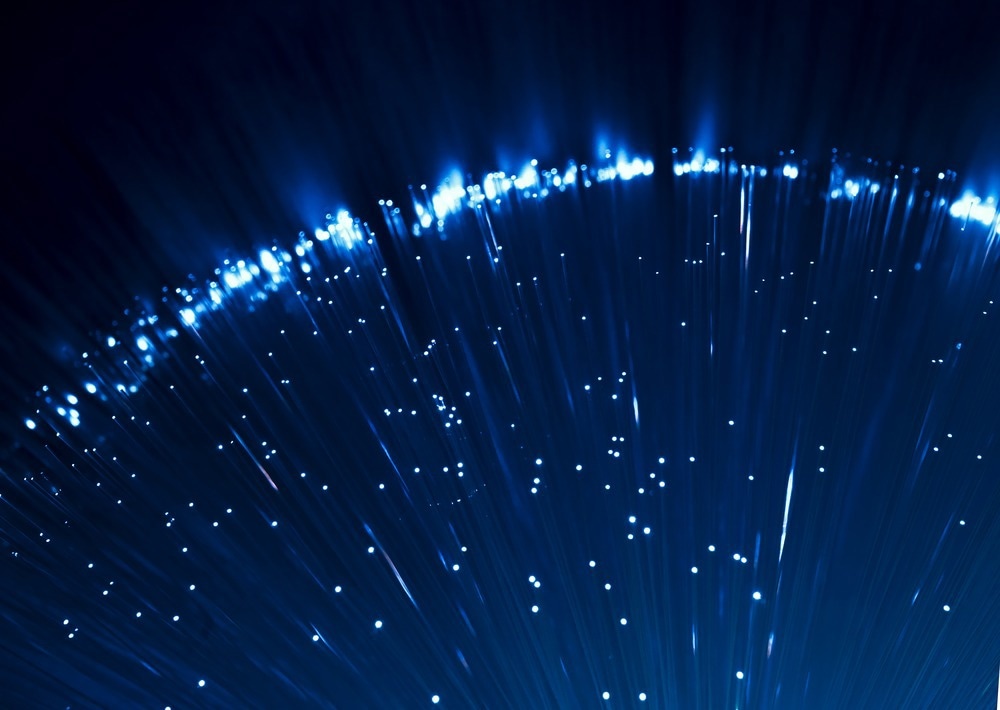
Image Credit: asharkyu/Shutterstock.com
The core innovation lies in the network's multi-synaptic connections, whose impact and robustness were evaluated through extensive numerical simulations. The network's resilience was tested against various configurations of synaptic weights and delay errors, showing an improvement in performance with multiple connections.
A practical demonstration involved a fabricated integrated distributed feedback laser with a saturable absorber (DFB-SA). This setup successfully classified ten distinct noisy digital patterns, showcasing the synergy between algorithmic computation and photonic hardware. The researchers employed time-division multiplexing to overcome hardware integration scale limits, enabling efficient photonic SNN operations beyond current hardware constraints.
This research marks the first application of multi-synaptic connections in photonic SNNs. By introducing multiple coupling paths between neurons, each with different efficacies and delays, the network's digit pattern classification capabilities were significantly enhanced. The findings suggest that these multi-synaptic connections can effectively boost network performance.
The advancement in photonic SNNs offers new possibilities in optical computing, particularly in learning algorithms and hardware integration. By leveraging the high speed and bandwidth of optical platforms, photonic SNNs represent a promising direction in overcoming the limitations of traditional microelectronic neurons in terms of energy efficiency and processing speed.
Source:
Han YN, Xiang SY, Song ZW, Gao S, Guo XX et al. Pattern recognition in multi-synaptic photonic spiking neural networks based on a DFB-SA chip. Opto-Electron Sci 2, 230021 (2023). doi: 10.29026/oes.2023.230021