Imaging weakly scattering phase objects, such as cells, has been a focus of research in a variety of domains, including biomedical sciences, for decades. To provide image contrast to weakly scattering materials, one frequent way involves chemical stains or fluorescent tags, however, this needs somewhat sophisticated sample preparation processes that can potentially be poisonous or damaging to samples.
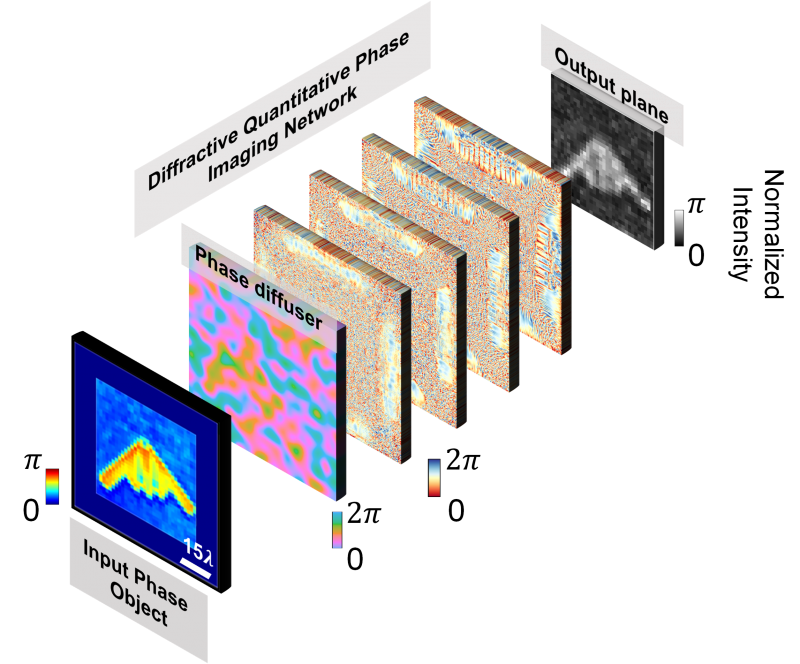
The schematic of the presented QPI diffractive network, which reveals the quantitative phase information of an object behind a random unknown diffuser without the need for a digital image reconstruction algorithm. Image Credit: Light: Advanced Manufacturing
Quantitative phase imaging (QPI) has emerged as an effective label-free solution to this problem, allowing for non-invasive, high-resolution imaging of transparent specimens without the need for any external chemicals.
Traditional QPI systems, on the other hand, might be resource-intensive and sluggish due to their reliance on digital image reconstruction and phase retrieval techniques. Furthermore, most QPI techniques fail to account for random scattering medium, which is notably common in biological tissue.
Researchers from the Electrical and Computer Engineering Department at the University of California, Los Angeles (UCLA), USA, recently reported a new method for quantitative phase imaging of objects that are entirely covered by random, unknown phase diffusers in a study that was recently published in Light: Advanced Manufacturing.
Their approach makes use of a diffractive optical network with an axial span of ~70 λ, where λ is the illumination wavelength, made up of consecutive transmissive layers that have been deep-learned-optimized.
Different randomly generated phase diffusers were used during training to help the system develop resistance to phase perturbations caused by random, unknown diffusers. Once trained, the diffractive layers produced can perform all-optical phase recovery and quantitative phase imaging of unidentified objects that are completely obscured by unidentified random diffusers.
Through new random phase diffusers that had never been seen before, the team’s numerical simulations effectively illustrated the QPI diffractive network’s ability to enable all-optical phase recovery and quantitative phase imaging of novel objects.
Further investigation into the effects of numerous variables, such as the number of diffractive layers and the trade-off between output energy efficiency and image clarity, revealed that deeper diffractive optical networks could often outperform shallower systems. Without rebuilding or retraining its layers, this QPI diffractive network can be physically scaled to function at various frequencies of the electromagnetic spectrum.
Low power consumption, high frame rate, and small size are advantages of such an all-optical computer system. The UCLA research group is looking forward to the potential integration of their QPI diffractive designs onto image sensor chips (CMOS/CCD imagers), effectively converting a conventional optical microscope into a diffractive QPI microscope capable of executing on-chip phase recovery and image reconstruction through light diffraction within passive structured layers.
Journal Reference:
Li, Y., et al. (2023) Quantitative phase imaging (QPI) through random diffusers using a diffractive optical network. Light: Advanced Manufacturing. doi:10.37188/lam.2023.017