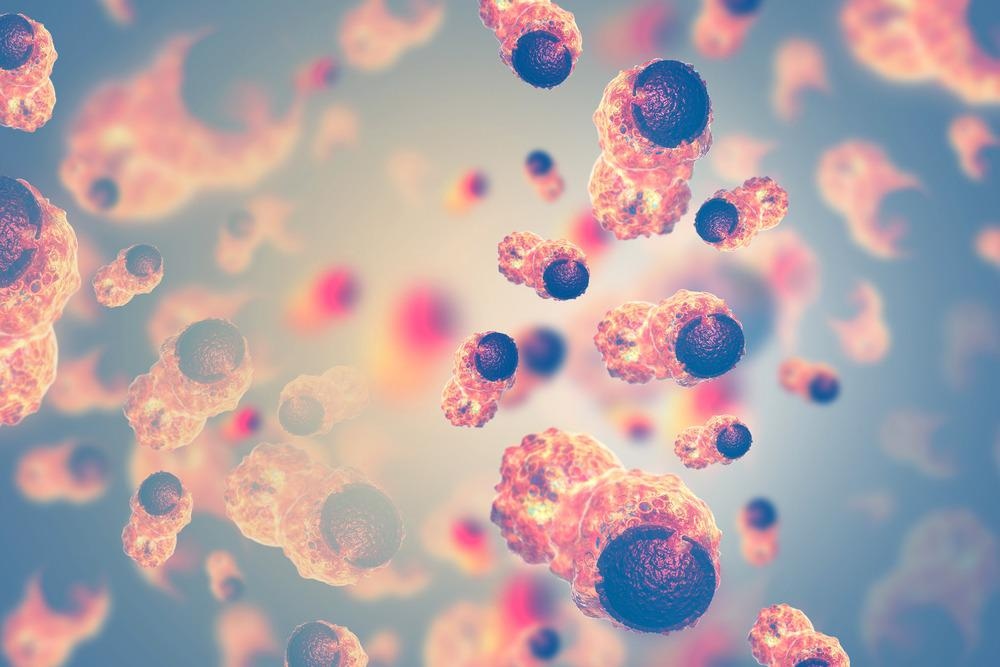
Image Credit: crystal light/Shutterstock.com
One of the key strategies in improving outcomes for cancer patients is earlier detection and diagnosis. This is why so many national strategies have focused on either screening or the involvement of primary care staff in both symptom recognition and as direct-access providers to diagnostic tools.
Just how quick can cancer diagnosis be? Often, a multi-pronged approach is taken to diagnosis. This may involve using non-invasive imaging techniques, such as X-rays and ultrasound, alongside tissue biopsies.
Imaging can often be a relatively quick process, with some procedures only needing a few minutes for image acquisition. However, for the majority of cancers, biopsies are still considered the preferred method for diagnosis and can take a number of days.
However, in ‘live’ situations like surgery, the time taken to process biopsy samples using optical microscopy techniques is simply too long to be feasible for online diagnosis. Tools to identify if all cancerous cells had been successfully removed or make a rapid diagnosis would be immensely beneficial for surgical settings.
To address this, there is a need for rapid imaging techniques that require minimal sample preparation and are straightforward to analyze.
Imaging techniques that can also recover spectroscopic information may hold the key to addressing all of these challenges. Recent developments in infrared spectroscopic imaging in combination with machine learning seem to offer some promising new tools for rapid diagnosis of cancers, including breast cancer.
Infrared Eyes
Infrared spectroscopy is sensitive to the different vibrational modes of a molecule or material. As the energies of those vibrational modes are dependent on the types of atoms and the strengths of the chemical bonds between them, the resulting infrared spectrum provides a powerful chemical fingerprint that can be used for both quantitative and qualitative analysis.
For medical imaging, infrared spectroscopy can be used to visualize changes at a molecular level, rather than looking only at the structural changes on the cells present in a biopsy sample. Many diseases result in changes in the molecular species found in the cell, which can be identified through the chemical fingerprinting capability of infrared spectroscopy.
The imaging information helps map which cells the spectroscopic information is being recorded from and the two sets of information can be combined for a fuller picture of what changes have occurred in the tissue.
Recent improvements in the spatial resolution of infrared imaging mean it is now feasible to achieve 2 µm pixel sizes for high-definition image acquisition and automated diagnosis in only an hour for epithelial cells.
This level of resolution for the study of cell histopathology starts to be at a competitive level with optical microscopy and the advantage of using infrared imaging is that spectral information can also be recovered on the cells of interest.
The latter is very important in enhancing our understanding of the chemical basis of cancer and identifying which compounds may be uniquely attributable to tumor species for the development of novel treatment methods.
Different Configurations
There are a variety of different microscope configurations that can be used for infrared imaging depending on what types of images are required. Standard definition microscopes typically have higher signal-to-noise due to higher throughput per pixel but compromise on the greater morphological details that can be resolved with high-definition experiments.
The question remains as to whether the additional morphological structure information from high-definition experiments is worth the additional analysis times for improving medical diagnoses.
For breast cancer, standard definition scans appeared to be sufficient for coarse patient-level statics, but with higher definition measurements it was possible to recognize and differentiate cell types and identify the disease state of the tissue.
Which resolution was optimal was somewhat situation-dependent – rapid diagnoses would favor the faster to acquire standard-resolution images whereas more detailed studies would require a higher-definition approach.
From this work, infrared imaging appears to be a sufficiently accurate tool for tissue histopathology with the added benefit of providing molecular data as well. Integration with machine learning approaches for the automated identification and classification of data also means that the speed of a diagnosis can be limited to the image acquisition and processing times.
Rapid and Early Diagnosis
The hope is that the chemical sensitivity and imaging capabilities of infrared imaging will make it a robust tool for early-stage diagnosis of cancer. Most imaging techniques can only be used in a diagnostic context when the tumor has grown sufficiently that visual changes can be observed. Long before such changes are observable, a cancerous site has already undergone significant genetic changes.
Early-stage diagnosis from cell histopathology alone is error-prone and highly dependent on the pathologist’s judgement. The ability to identify chemical biomarkers associated with early-stage tumor formation, which would have different chemical fingerprints in their infrared spectra, would provide another dimension of information for diagnosis as well as objective criteria (i.e. the presence of infrared bands associated with cancerous tissue) as opposed to more subjective interpretation relying on cell structures.
Improvement in optics, microscope throughput and scattering suppression schemes will all play an important role in helping to acquire better quality infrared images. For the data analysis, chemometrics techniques used widely in analytical chemistry may help with the automated identification of the chemical composition of cells, while image recognition methods can help diagnosis through cell histopathology.
References and Further Reading
Rubin, G., Vedsted, P., & Emery, J. (2011). Improving cancer outcomes: Better access to diagnostics in primary care could be critical. British Journal of General Practice, 61(586), 317–318. https://doi.org/10.3399/bjgp11X572283
Mittal, S., Wrobel, T. P., Walsh, M., Kajdacsy-Balla, A., & Bhargava, R. (2021). Breast cancer histopathology using infrared spectroscopic imaging: The impact of instrumental configurations. Clinical Spectroscopy, 3(July 2020), 100006. https://doi.org/10.1016/j.clispe.2021.100006
Su, K. Y., & Lee, W. L. (2020). Fourier transform infrared spectroscopy as a cancer screening and diagnostic tool: A review and prospects. Cancers, 12(1). https://doi.org/10.3390/cancers12010115
Khanmohammadi, M., Bagheri Garmarudi, A., Samani, S., Ghasemi, K., & Ashuri, A. (2011). Application of linear discriminant analysis and attenuated total reflectance fourier transform infrared microspectroscopy for diagnosis of colon cancer. Pathology and Oncology Research, 17(2), 435–441. https://doi.org/10.1007/s12253-010-9326-y
Disclaimer: The views expressed here are those of the author expressed in their private capacity and do not necessarily represent the views of AZoM.com Limited T/A AZoNetwork the owner and operator of this website. This disclaimer forms part of the Terms and conditions of use of this website.