In several scientific disciplines, such as biochemistry, physiology, and neuroscience, microscopes are crucial instruments for scientific study. To rectify light wavefront aberrations caused by intrinsic optical system limits and non-uniform sample structures, adaptive optics, or AO, have been employed in microscopes. Sample structures and wavefront aberrations were unknown before imaging, making AO difficult to regulate even though it significantly improves microscope imaging quality.
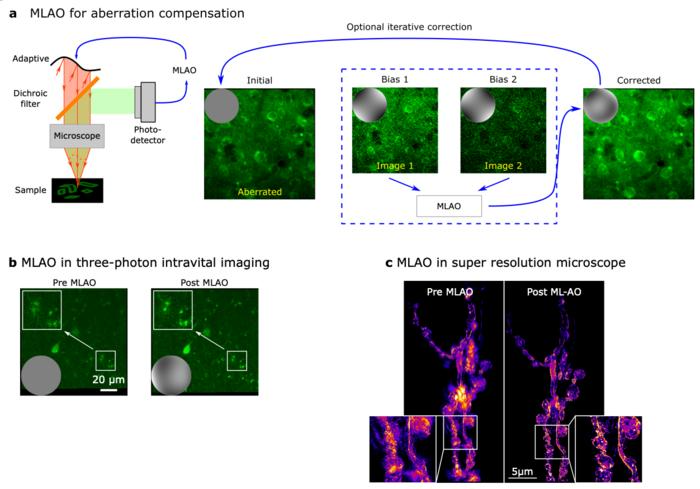
(a) MLAO was embedded in the microscope control to improve imaging quality by modulate the illumination light. Right-hand side showed an example of one operation cycle using MLAO. After using MLAO with two sample exposures, the imaging quality was largely improved as the image labelled as corrected was much brighter and sharper than the image labelled as initial. (b) MLAO was used in three-photon intravital deep tissue imaging of a mouse brain. After MLAO, the cells were brighter, sharper and more recognisable. (c) MLAO was used in super-resolution imaging of Drosophila larval neuromuscular junctions. After MLAO, the image was much sharper with a lot more fine features resolved and hexagonal artefacts removed. Image Credit: Qi Hu, Martin Hailstone, Jingyu Wang, Matthew Wincott, Danail Stoychev, Huriye Atilgan, Dalia Gala, Tai Chaiamarit, Richard M. Parton, Jacopo Antonello, Adam M. Packer, Ilan Davis & Martin J. Booth
Additional wavefront sensors are frequently needed for conventional AO systems, which can be expensive and time-consuming. Suggested sensorless methods typically call for iterative rectification procedures, which causes sample photodamage. As of yet, no single approach exists that is simple to use and adaptable for numerous microscopy modes.
A team of Oxford researchers created a machine learning-based AO (MLAO) technique to integrate AO into the microscope imaging process and significantly enhance imaging quality, as described in the cover article of Light: Science & Application issue 12.
Dr. Qi Hu, a Schmidt AI in science fellow, oversaw the study under the direction of Prof. Martin Booth, both of the University of Oxford’s Department of Engineering Science. This novel technique used fewer sample exposures than traditional AO approaches and showed resilience in the face of many difficult imaging tasks, such as managing high noise levels, random sample motions, and “blinking” occurrences in functional imaging.
A two-photon, three-photon, and widefield three-dimensional structured illumination super-resolution microscope was used to illustrate the technique. In contrast to other machine learning-based techniques, this method presented a custom convolutional neural network (CNN) architecture that was orders of magnitude smaller than other CNN structures that are frequently utilized.
It also encapsulated physical understandings. Additionally, the physical aspects of the imaging process were included in this custom-developed CNN.
The study authors stated, “This means that the internal CNN configuration needs no longer to be considered as a “black box,” but can be used to provide physical insights on internal workings and how information about aberrations is encoded into images.”
A Universal Method That Can Be Implemented in Different Microscope Modalities
Unlike earlier approaches that typically needed a particular optical design or were restricted to specific microscope modalities, this suggested strategy was shown to be adaptable to various microscopes. The same construction can be used even though separate training datasets must be created to accommodate various physical models of imaging equipment.
Additionally, when the system was trained for various imaging applications, the CNN architecture remained unaltered. The research demonstrated the technique using a two-photon, three-photon, and widefield three-dimensional structured illumination microscope, demonstrating its outstanding versatility and broad applicability.
A fair comparison between the MLAO approach and its traditional equivalents was made. According to statistical findings, the MLAO method generally performed better than the traditional methods by correcting with fewer sample exposures. The MLAO approach outperformed the traditional methods in a limited number of circumstances. Additionally, the MLAO approach worked more reliably and consistently.
Additionally, it was shown that the MLAO method worked well for difficult imaging applications where traditional methods usually fail, like functional dynamic imaging and live imaging with random sample motions. It was also demonstrated that MLAO possessed the beneficial property of being resilient against changes in noise level, imaging sampling rate, and out-of-focus structures.
Physical understandings of the imaging process were specifically captured in the CNN architecture employed in the MLAO algorithm. By connecting CNN weights to physical characteristics, it is possible to analyze not only the effectiveness of the MLAO algorithm but also its advantages and disadvantages. Additionally, the design of future methods can benefit from this special characteristic.
Owing to its adaptability and efficiency, the MLAO approach holds significant promise for microscopy imaging applications and can be beneficial for a variety of scientific study domains, such as neuroscience, physiology, biochemistry, and ophthalmology.
Journal Reference
Hu, Q., et al. (2023) Universal adaptive optics for microscopy through embedded neural network control. Light: Science & Application. doi:10.1038/s41377-023-01297-x.