What happens when a 2021 algorithm-driven microscopy technology, capable of working with a smaller subset of images compared to previous methods, isn't fast enough? Delving deeper and squaring the solution - this is precisely what Dushan Wadduwage, a John Harvard Distinguished Science Fellow at the FAS Center for Advanced Imaging, did.
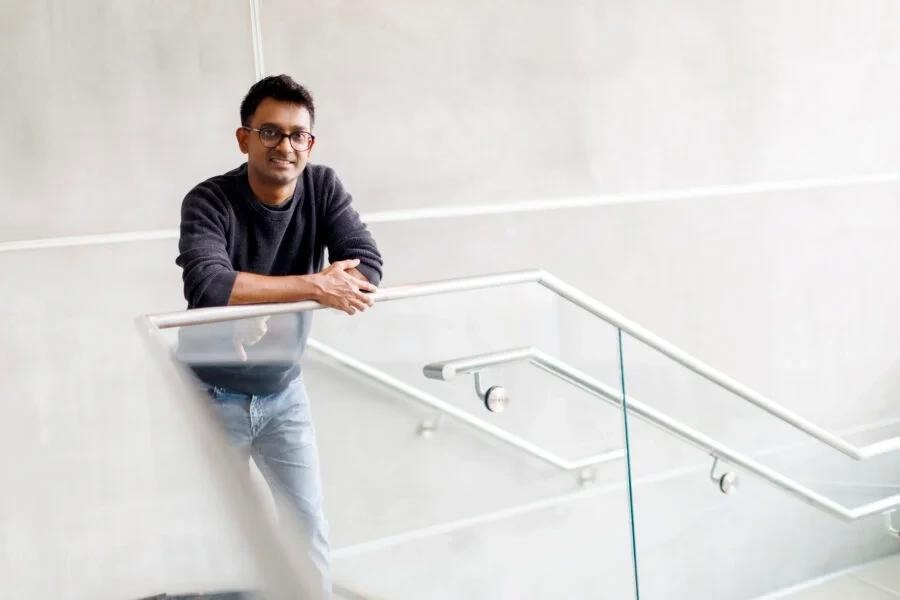
Dushan Wadduwage. Image Credit: Stephanie Mitchell/Harvard Staff Photographer
For decades, scientists have attempted to visualize the depths of a living brain. They began by experimenting with fluorescence microscopy, a century-old technology that uses fluorescent molecules and light. However, the wavelengths were insufficiently long, and they dispersed before reaching a significant distance.
When two-photon microscopy was invented in 1990, it allowed longer wavelengths of light to shine onto tissue, allowing fluorescent molecules to absorb not one but two photons. Longer wavelengths were employed to stimulate molecules, which scattered less and could penetrate further.
However, two-photon microscopy can often only stimulate one location on the tissue at a time, resulting in a lengthy procedure needing several observations. A speedier method of imaging would be to illuminate numerous spots at once with a broader field of view, but this, too, had problems.
If you excite multiple points at the same time, then you can’t resolve them. When it comes out, all the light is scattered, and you don’t know where it comes from.
Dushan Wadduwage, John Harvard Distinguished Science Fellow, FAS Center for Advanced Imaging, Harvard University
Wadduwage’s group began employing a specific type of microscopy to address this obstacle, which was published in Science Advances in 2021. Using distinct pre-encoded excitation patterns, the scientists activated numerous spots on the tissue in a wide-field mode. This technology, known as De-scattering with Excitation Patterning, or DEEP, is powered by a computational algorithm.
“The idea is that we use multiple excitation codes, or multiple patterns to excite, and we detect multiple images. We can then use the information about the excitation patterns and the detected images and computationally reconstruct a clean image,” Wadduwage added.
The quality of images obtained is equivalent to that of point-scanning two-photon microscopy. They can still be created with hundreds of images rather than the hundreds of thousands required for point-scanning. Wadduwage’s team was able to examine as far as 300 microns into live mouse brains using the new technology.
Still insufficient. Wadduwage wondered if DEEP might provide a clear image with only a few tens of images.
He used machine learning to speed up the imaging process in a new study published in Light: Science and Applications. He and his colleagues employed artificial intelligence to train a neural network-driven algorithm on repeated sets of images, finally training it to rebuild a properly resolved image with just 32 dispersed images (rather than the 256 stated in their original study). DEEP-squared is a deep learning-powered de-scattering approach with excitation patterning.
The researchers used images from standard two-photon point-scanning microscopy to provide what Wadduwage referred to as the “ground-truth.” The DEEP microscope then employed physics to create a computational model of the image generation process, which it then used to simulate dispersed input images.
These were used to train their DEEP-squared AI model. The researchers employed AI to take new images of blood vessels in a mouse brain after it created rebuilt images that mirrored Wadduwage’s ground-truth reference.
Wadduwage further stated, “It is like a step-by-step process. In the first paper we worked on the optics side and reached a good working state, and in the second paper we worked on the algorithm side and tried to push the boundary all the way and understand the limits. We now have a better understanding that this is probably the best we can do with the current data we acquire.”
Nonetheless, Wadduwage has further suggestions for expanding DEEP-squared’s capabilities, such as upgrading equipment design to capture data faster. He believes DEEP-squared demonstrates cross-disciplinary collaboration, as would any future technological advancements.
Wadduwage concluded, “Biologists who did the animal experiments, physicists who built the optics, and computer scientists who developed the algorithms all came together to build one solution.”
Journal Reference:
Wijethilake, N., et al. (2023) DEEP-squared: deep learning powered De-scattering with Excitation Patterning. Light: Science and Applications. doi:10.1038/s41377-023-01248-6