Deep learning-based landslide detection methods are highly efficient. A recent study published in Remote Sensing proposes the landslide detection model YOLOX-Pro based on the enhanced YOLOX (You Only Look Once) target detection model to improve the deficient detection of complex landslides.
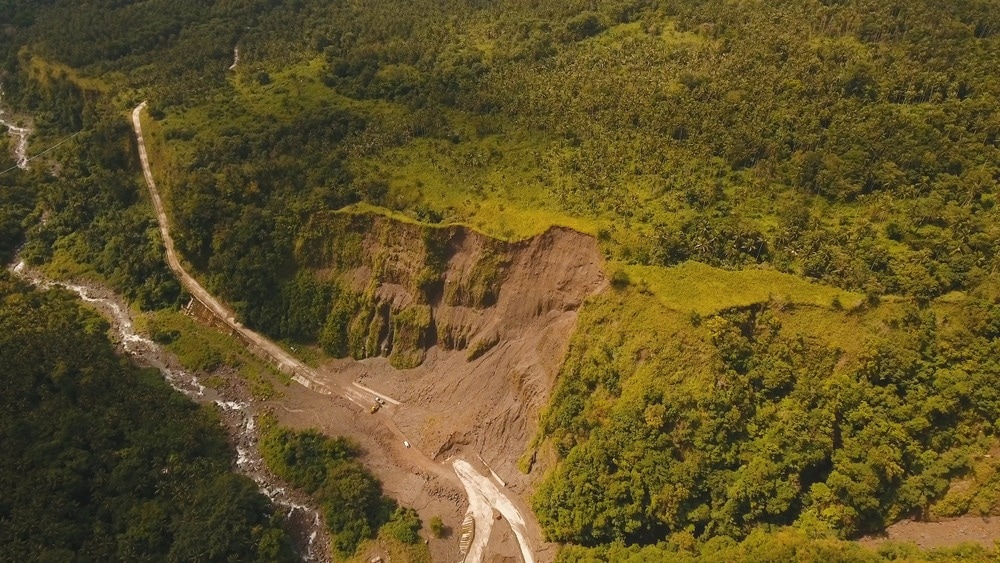
Study: A Universal Landslide Detection Method in Optical Remote Sensing Images Based on Improved YOLOX. Image Credit: Alex Traveler/Shutterstock.com
The VariFocal was used in place of the binary cross entropy to address the unequal distribution of landslide samples and enhance detection recall. A total of 1200 historical landslide optical remote sensing photos in 38 regions in China were taken from Google Earth to produce a mixed sample set for landslide identification.
Dangers of Landslides
Landslides are geological disasters that critically risk human life, infrastructure, and the environment. Global climate change increases the frequency and severity of landslide disasters. A dammed lake formed as a result of the 2018 Baige landslide in the Tibet river caused catastrophes hundreds of kilometers downstream.
Satellite images are vital for timely disaster analysis for discovering and gathering data on landslide feature information for landslide catastrophes happening in uninhabited areas.
Limitations of Traditional Landslides Detection Methods
Traditional detection of landslide hazards encompasses field investigation and human visual interpretation techniques using remote sensing data. The conventional approaches are reliable but take a lot of time and effort and rely on specialist knowledge. The object-based thresholding method statistically examines a landslide's particular textural, spectral, or geomorphological properties to determine one or more thresholds for landslide detection. However, the threshold method has limited application and poor generalization because the feature threshold is determined according to the particular research area. The detection effect decreases when the research area changes.
The change detection approach based on two-dimensional optical pictures or three-dimensional topography data for two or more periods is used for detecting changes in landslide areas. Fresh landslides respond better to change detection, but this method necessitates time series remote sensing data. The most popular optical image-based machine learning detection techniques include vector machine (SVM), random forest (RF), and artificial neural network (ANN). These techniques involve additional work in obtaining image feature data, troubleshooting hyperparameters, and running more feature selection tests.
Convolutional Neural Networks (CNN)-Based Algorithms for Landslide Detection
Deep learning technology has advanced swiftly in recent years. Convolutional neural networks (CNN)-based detection algorithms have achieved vast success in semantic segmentation, image classification, and object identification. Convolutional neural networks can process complicated images and automatically extract multi-layer characteristics from the original image efficiently.
One-stage and two-stage target detection techniques based on CNN are obtainable. The end-to-end detection category includes the one-stage algorithms of the YOLOX series with increased detection speed. Two-stage R-CNN algorithms have significantly slower detection speeds but improved detection precision. The two methods mentioned above have emerged as research hotspots in landslide detection for identifying the automatic detection of landslides in a specific area.
However, the majority of the research currently in existence is focused on landslide detection in particular places, with insufficient robustness and accuracy for landslide detection in numerous categories in various terrains and landscapes.
Development of Universal Method (YOLOX-Pro) For Lanslide Detection
Hou et al. suggested the YOLOX-Pro technique based on the enhanced YOLOX method for landslide detection in optical remote sensing images. YOLOX-Pro increases the precision for landslide detection in various geomorphological settings. YOLOX-Pro can detect both simple and complicated landslides.
The researchers used the varifocal loss function to address miss detection and poor accuracy for minuscule landslides. The attention mechanism was also included to enhance the capability of landslide detection sites. YOLOX-Pro detection performance involved comparing YOLOX-Pro with four object detection models by testing the impact of three attention mechanisms.
Research Findings
This research established a dataset containing multiple types of remote sensing landslides using Google Earth images as the data source. A set of YOLOX-Pro networks based on the deep learning model YOLOX was constructed by replacing the VariFocal loss function and introducing a lightweight Coordinate Attention mechanism. YOLOX-Pro was used to develop a universal detection method for multi-category landslides.
YOLOX-pro was used by selecting a network of the corresponding size according to the detection equipment’s storage and computational capacity status, which has broad application prospects. To evaluate the capability of YOLOX-Pro in detail, the COCO evaluation metrics were introduced into the landslide detection task.
The results showed that the proposed method has high detection accuracy for small and complex mixed landslides. The detection results of the group-occurring landslide area and the UAV landslide images verified the robust performance of the YOLOX-Pro model. The researchers also discussed the limitations and challenges faced by YOLOX-Pro, which provided a technical reference for further exploration of landslide hazard research using deep learning techniques.
In the future, the use of semantic segmentation algorithms to obtain more information about landslides for hazard surveys and explore deploying lightweight models to embedded devices to detect landslides automatically can be investigated.
References
Hou, H., Chen, M., Tie, Y., & Li, W. (2022). A Universal Landslide Detection Method in Optical Remote Sensing Images Based on Improved YOLOX. Remote Sensing, 14(19), Article 19. https://www.mdpi.com/2072-4292/14/19/4939
Disclaimer: The views expressed here are those of the author expressed in their private capacity and do not necessarily represent the views of AZoM.com Limited T/A AZoNetwork the owner and operator of this website. This disclaimer forms part of the Terms and conditions of use of this website.