The combined effect of forward scattering and defocus blurs underwater imaging and reduces its quality. To address this, a study published in Photonics has proposed a two-step underwater image deblurring method. It enhances the image quality by removing backscattered light and deblurs the image via a deep learning model.
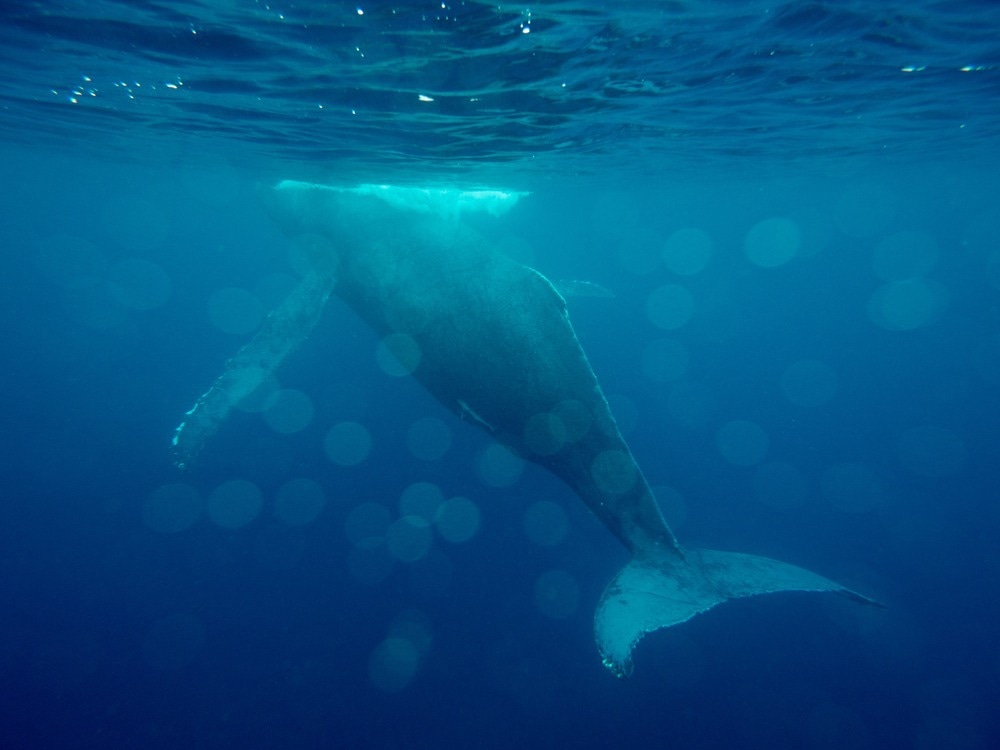
Study: Single Image Deblurring for Pulsed Laser Range-Gated Imaging System with Multi-Slice Integration. Image Credit: GAO5555/Shutterstock.com
Blurring in Underwater Imaging System
Pulsed laser range-gated imaging (PLRGI) is an efficient technology for obtaining high-resolution underwater images. However, the conventional PLRGI method is mostly utilized for in situ observation with a limited depth of view (DOV).
Multi-slice integration (MSI) is a common method used to increase the DOV of the PLRGI system. However, when the DOV is large and surpasses the system's depth of field, it causes some targets in the image to be blurred.
Forward Scattering
The blurring of an underwater image is also affected by the forward scattering of water. It alters the light's trajectory and blurs the image. Various solutions have been developed to mitigate the blurring induced by forward scattering.
One method is to create a model based on the target's form and backdrop and then use it to predict the distribution and intensity of forward scattering light. However, it is a sensitive, unstable, and inaccurate method, as estimating underwater imaging parameters is challenging.
Another option is to iteratively deconvolve the blurry image; however, this approach has a high computational cost.
Defocus Blur
The conventional method for removing defocus blur involves estimating the point spread function and then applying a deconvolution technique to recover a clear image. However, the point spread function is hard to estimate as the underwater environment is complex and underwater imaging models can be inaccurate.
Researchers have preferred deep learning algorithms in recent years to reduce defocus blur. This method employs an end-to-end network design, which avoids various modeling issues and allows for direct mapping of blurry images to clear ones.
However, these approaches are based on convolutional neural networks, which only simulate the link between adjacent pixels.
A blurred image is generated by the combined effect of defocus and forward scatter in underwater range-gated imaging. However, the majority of the research focuses on eliminating forward scattering. There is a lack of research that addresses the combined effects of defocus blur and forward scatter because of the small depth of view of the PLRGI system.
Deblurring Underwater Imaging by Deep Learning and Rolling Ball Method
This study proposes a two-step approach to deblur an image that has been blurred by the combined action of forward scatter and defocus.
The proposed method was created using the Jaffe-McGlamery and Fourier optics models. The imaging model states that forward scatter is associated with the reflected light, whereas backscattered light is independent of reflected light from the target. So, before deblurring, backscattered light should be eliminated.
Therefore, the image was first enhanced by using the rolling ball and intensity modification to eliminate backscattered light. It was then deblurred using a Transformer based deep learning model.
The deep learning model was trained using an imaging model, which enables the model to handle varying degrees of visual blurriness. The imaging model was used to create 16 alternative blur kernels, allowing the deep learning model to tolerate various levels of image blurriness.
Images with varying degrees of blurriness were gathered from a boat tank and a water tank by the PLRGI system using an MSI approach as test sets for verifying the proposed method.
Important Findings of the Study
Various experiments were conducted under multiple water conditions, and the findings indicate that the proposed method is reliable and efficient. The suggested approach can simultaneously handle images that contain clear and blurry targets and can remove varying levels of blur.
The proposed method was compared against two state-of-the-art defocus deblurring methods: kernel-sharing parallel atrous convolutional (KPAC) and Gaussian kernel mixture deep neural network (GKMNet).
It outperformed both methods in various experiments under different conditions in terms of both quantitative metrics and visual quality. Furthermore, the proposed two-step method generates high quality sharper images, whereas KPAC suffers from significant noise issues and GKMNet has no significant impact on large-scale blur.
Despite the high deblurring performance, the deep learning model based on Transformer cannot handle this task in real-time due to its lengthy processing time. Future research will address this issue to implement the proposed model in a practical compact system.
Reference
Lin, H., Ma, L., Hu, Q., Zhang, X., Xiong, Z., & Han, H. (2022). Single Image Deblurring for Pulsed Laser Range-Gated Imaging System with Multi-Slice Integration. Photonics. https://www.mdpi.com/2304-6732/9/9/642/htm
Disclaimer: The views expressed here are those of the author expressed in their private capacity and do not necessarily represent the views of AZoM.com Limited T/A AZoNetwork the owner and operator of this website. This disclaimer forms part of the Terms and conditions of use of this website.