In a pre-proof study from the Journal of Quantitative Spectroscopy and Radiative Transfer, researchers used artificial intelligence (AI) models to effectively predict the emissivity of functionalized surfaces produced by the femtosecond laser surface processing method.
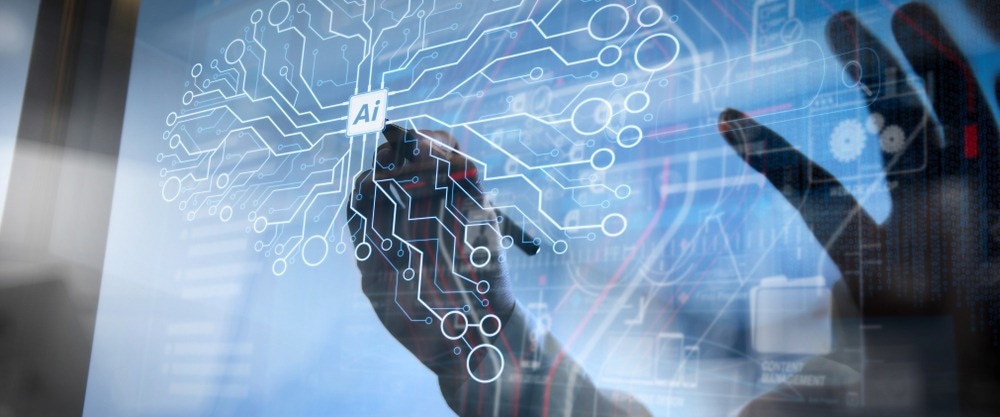
Study: Emissivity Prediction of Functionalized Surfaces Using Artificial Intelligence. Image Credit: everything possible/Shutterstock.com
Due to the surface's contributing variables, dependency, complex interactions, and interconnectedness, it is challenging to estimate the surface emissivity using a physics-based modeling technique. As a result, a new strategy is required to accurately predict emissivity and broaden the scope of thermal radiation's potential applications.
Significance of Surface Emissivity
The surface emissivity of a material is measured as the ratio of the energy emitted from its surface to the energy emitted from a perfect emitter (black body).
Every material above absolute zero temperature emits thermal radiation. However, the amount of thermal radiation emitted for any given wavelength and temperature varies on the surface emissivity of the material.
The surface emissivity can be tuned to increase the emissivity of the material. Tuning surface emissivity plays a key role in thermal radiation applications, such as passive radiative cooling, thermal management systems, and thermophotovoltaics.
How Can Surface Emissivity Be Tuned?
Coatings and paints can alter the surface emissivity of a material. Their adaptability to large or curved surfaces has led to their applications in radiation pyrometry, thermal imaging, and thermophotovoltaic emitters. However, susceptibility to wear and lamination to changes in climatic conditions has limited their applications.
Metamaterials production has been pursued to achieve surfaces with adequate directional and spectral emissivity. However, their output is often restricted to a narrow spectral region and needs a costly micro-manufacturing process.
Using FLSP to Manufacture Surfaces with Desired Emissivity
Femtosecond laser surface processing is used to create functionalized surfaces with desired emissivities. It is a cost-effective and straightforward production process.
FLSP surfaces exhibit a notably wider bandwidth response, excellent permanence, and durability even in harsh environments.
Despite the adaptability of the FLSP method and technological developments in fabrication techniques for engineered surfaces, there is still a substantial issue in predicting the emissivity before fabrication. An accurate prediction of surface emissivity can greatly reduce the time and effort required for manufacturing.
Implementation of Artificial Intelligence to Predict Surface Emissivity
Due to the complexity of physics-based model-driven approaches, the pre-proof study published in the Journal of Quantitative Spectroscopy and Radiative Transfer has employed artificial intelligence techniques to predict the emissivity of functionalized surfaces.
The surface properties of bulk samples of the aluminum alloy 6061 were adjusted with the femtosecond laser surface processing manufacturing technique in a well-controlled environment.
Laser scanning confocal microscopy with a 50x magnification recorded the 3D topography in three distinct regions of each sample to thoroughly analyze the FLSP samples' surface features.
In this study, 116 distinct samples were characterized, fabricated and tested to compile a database of laser operating parameters and measure hemispherical emissivity and surface attributes.
The measured hemispherical emissivity and the entire image dataset helped artificial intelligence estimate the emissivity of a fresh FLSP sample.
The derived features from the LSCM images were coupled with the laser operating parameters and surface characteristics to determine whether artificial intelligence can accurately predict the emissivity based on femtosecond laser surface processing parameters and surface characteristics.
A convolutional neural network (CNN) was pre-trained to function as a feature extractor on the training image dataset. An artificial neural network (ANN) was created to divide the test samples into seven emissivity groups.
Important Findings of the Study
The combination of deep learning and machine learning reliably predicts the emissivity of FLSP samples based on their fabrication parameters and surface properties.
Artificial intelligence techniques can predict the specific emissivity range for a surface using its 3D morphological image.
According to the results, artificial intelligence-based image processing algorithms have a significant advantage over physics-based models in calculating emissivity.
Artificial neural network (ANN) and decision tree (DT) models surpassed other machine-learning classifiers with estimated errors of 3.88% and 3.31%, respectively.
Despite a notable inaccuracy for two samples, artificial intelligence models accurately predicted the overall hemispherical emissivity of femtosecond laser surface processing functionalized surfaces.
Data-driven models can only be used within their training domains and cannot be used outside the training dataset. The artificial intelligence model must be retrained on the appropriate dataset to produce a data-driven predictive model for any additional material.
The successful performance of these artificial intelligence data-driven models will pave the way for predicting physical characteristics that would be impossible to anticipate using traditional physics-based modeling or may need extensive experimentation.
Reference
Acosta, G., Reicks, A., Moreno, M., Borjali, A., Zuhlke, C., & Ghashami, M. (2022) Emissivity Prediction of Functionalized Surfaces Using Artificial Intelligence, Journal of Quantitative Spectroscopy & Radiative Transfer. https://www.sciencedirect.com/science/article/abs/pii/S0022407322002606
Disclaimer: The views expressed here are those of the author expressed in their private capacity and do not necessarily represent the views of AZoM.com Limited T/A AZoNetwork the owner and operator of this website. This disclaimer forms part of the Terms and conditions of use of this website.