Current diagnostic methods for bacterial identification are time-intensive and require culturing, taking hours to days to complete. However, a recent study published in Nano Letters proposed a novel technique that combines Raman spectroscopy, acoustic bioprinting, and machine learning for rapid bacterial identification. This innovative technique promises improved clinical diagnosis, safer food, faster drug development, and enhanced environmental monitoring.
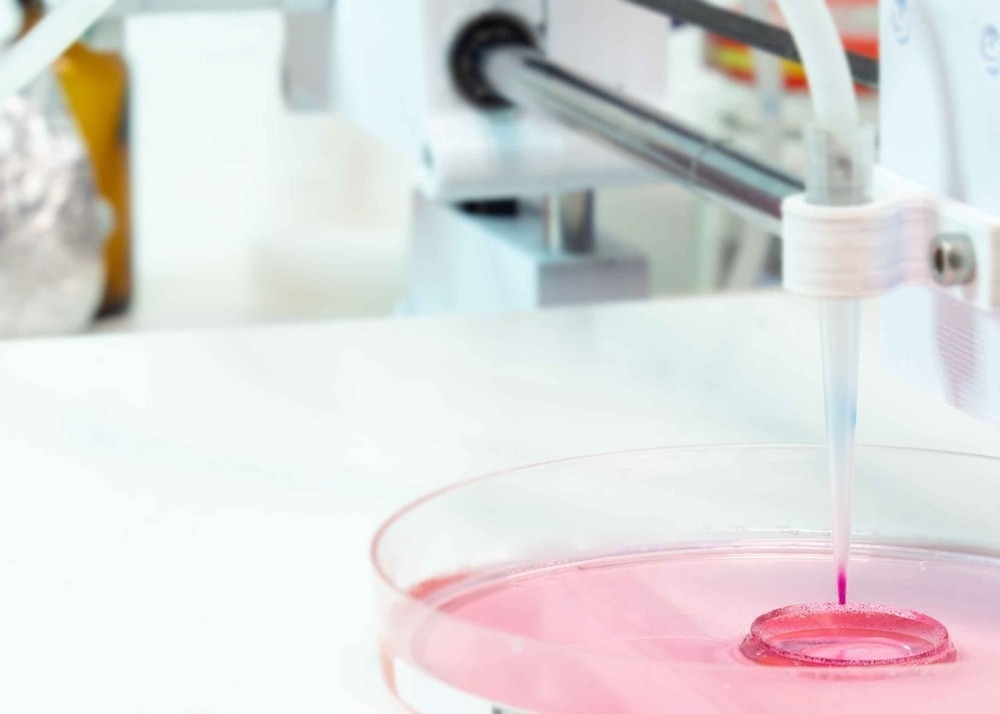
Image Credit: luchschenF/Shutterstock.com
Challenges and Demand for Rapid and Accurate Bacterial Identification
Bacterial infections are a major cause of death worldwide, leading to more than 7.7 million deaths annually.
The current diagnostic process involves culturing bacterial samples, which can take several days, and prescribing broad-spectrum antibiotics while waiting for results. Unfortunately, this leads to over 30% of patients being treated unnecessarily and contributes to developing antimicrobial resistance.
Improved analytical methods such as PCR techniques and immunological assays have been developed to shorten the total assay time with high specificity. However, they are limited in on-site testing and require expensive reagents.
As a result, there is a high demand for alternative methods to improve testing efficiency, especially for foodborne pathogens and clinical settings where rapid identification is critical for diagnosing the infection source.
What is Raman spectroscopy, and How is it Used for Bacterial Identification?
Raman spectroscopy is a label-free, non-invasive method of identifying bacterial species. This technique takes advantage of the unique molecular structure of each type and cell strain, giving rise to a distinct spectral fingerprint that can be used for identification.
By illuminating a sample with laser light, Raman spectroscopy measures the energy scattered (weak Raman scattering) during interactions between the light and the sample molecules. Then, it filters out the laser light to analyze the captured signal and match it with known bacteria.
What Advantages Does It Offer Over Other Diagnostic Methods for Bacterial Identification?
Compared to other nucleic-acid-based (polymerase chain reaction) or protein-based tests (enzyme-linked immunoassay) for bacterial identification, Raman spectroscopy offers several advantages, including low reagent usage, minimal sample preparation, low-cost equipment, non-destructive analysis, and the potential for amplification-free detection.
The development of surface-enhanced Raman spectroscopy has further increased the accessibility of this technique for portable and low-cost diagnostics, making it an attractive option for bacterial identification in food safety, clinical diagnosis, and environmental monitoring.
Limitations
Although Raman spectroscopy has shown great promise for identifying bacterial species, its effectiveness can be hindered by low signal-to-noise ratios and the need for extensive datasets to cover many relevant bacterial strains and antibiotic resistance patterns.
The method is limited by its sensitivity, accuracy, and susceptibility to interference from complex samples, such as clinical and food specimens. In addition, high laser power can potentially affect the bacterial structure and accuracy of the analysis.
To address these limitations, researchers are exploring using novel nanomaterials, more efficient bacterial separation methods, and advanced data processing tools to increase sensitivity and improve the analysis of raw spectral data.
New AI-Assisted Method Combining Raman Spectroscopy and Acoustic Bioprinting Offers Rapid Bacterial Identification
It is challenging to distinguish bacteria from other molecules or cells in a given sample because they all exhibit unique light patterns. For example, even in a small amount of blood, there could be billions of cells, but only a few might be bacteria.
To address this challenge, researchers from Stanford University have proposed a new method to identify bacteria in fluids using a combination of surface-enhanced Raman spectroscopy, acoustic bioprinting, and machine learning. The results are published in Nano Letters.
The researchers used the acoustic droplet ejection (ADE) technique to isolate the cells in extremely small samples and to eliminate unwanted spectral information. ADE uses ultrasonic waves that create radiation pressure to eject a droplet from the surface, which is only a few dozen-sized cells.
The team added gold nanorods to the samples, which bind to bacteria and intensified the Raman signal by 1500 times. They then employed machine learning to compare the different light patterns emitted by each printed fluid dot to identify the unique Raman spectral signatures of any bacteria in the sample.
Significance of the Study and Future Outlooks
The proposed method offers a high throughput and a potential for rapid and affordable on-site diagnosis without requiring laboratory analysis, making it suitable for untrained personnel. In addition, it could lead to rapid, more accurate, and inexpensive microbial assays of many different fluids as an alternative to traditional culturing methods that can take hours or days.
This innovative method could contribute to Raman-based research, disease management, and clinical diagnostics. It may also enable the development of point-of-care systems for detecting biomarkers in body fluids with minimal invasiveness.
Although the technique was developed and refined using blood samples, the researchers are optimistic that it can be extended to examining other types of fluids and cells, such as testing drinking water purity.
It's an innovative solution with the potential for life-saving impact. We are now excited for commercialization opportunities that can help redefine the standard of bacterial detection and single-cell characterization.
Amr Saleh, Senior Co-Author of the Study
Further development of advanced data processing methods, a universal database, and simple sample preparation techniques are necessary to improve Raman spectra interpretation and create a rapid and portable bacterial identification system for in-field testing.
More from AZoOptics: 3D and 4D Machine Vision Solutions from SiLC
References and Further Reading
Safir, F., Vu, N., Tadesse, L. F., Firouzi, K., Banaei, N., Jeffrey, S. S., ... & Dionne, J. A. (2023). Combining Acoustic Bioprinting with AI-Assisted Raman Spectroscopy for High-Throughput Identification of Bacteria in Blood. Nano Letters. https://doi.org/10.1021/acs.nanolett.2c03015
Myers, A. (2023). Stanford Researchers Develop a New Way to Identify Bacteria in Fluids. [Online]. Stanford News. Available at: https://news.stanford.edu/press-releases/2023/03/02/new-way-identify-bacteria-fluids/
Ho, C. S., Jean, N., Hogan, C. A., Blackmon, L., Jeffrey, S. S., Holodniy, M., ... & Dionne, J. (2019). Rapid identification of Pathogenic Bacteria using Raman Spectroscopy and Deep Learning. Nature communications. https://doi.org/10.1038/s41467-019-12898-9
Rodriguez, L., Zhang, Z., & Wang, D. (2023). Recent Advances of Raman Spectroscopy for the Analysis of Bacteria. Analytical Science Advances. https://doi.org/10.1002/ansa.202200066
Tadesse, L. F., Ho, C. S., Chen, D. H., Arami, H., Banaei, N., Gambhir, S. S., ... & Dionne, J. (2020). Plasmonic and Electrostatic Interactions Enable Uniformly Enhanced Liquid Bacterial Surface-Enhanced Raman Scattering (SERS). Nano letters. https://doi.org/10.1021/acs.nanolett.0c03189
Disclaimer: The views expressed here are those of the author expressed in their private capacity and do not necessarily represent the views of AZoM.com Limited T/A AZoNetwork the owner and operator of this website. This disclaimer forms part of the Terms and conditions of use of this website.