A new theoretical study suggests building an optical neural network architecture for deep learning using the concept of a temporal synthetic dimension. The synthetic dimensions are created by the arrival times of optical pulses in a single-ring resonator.
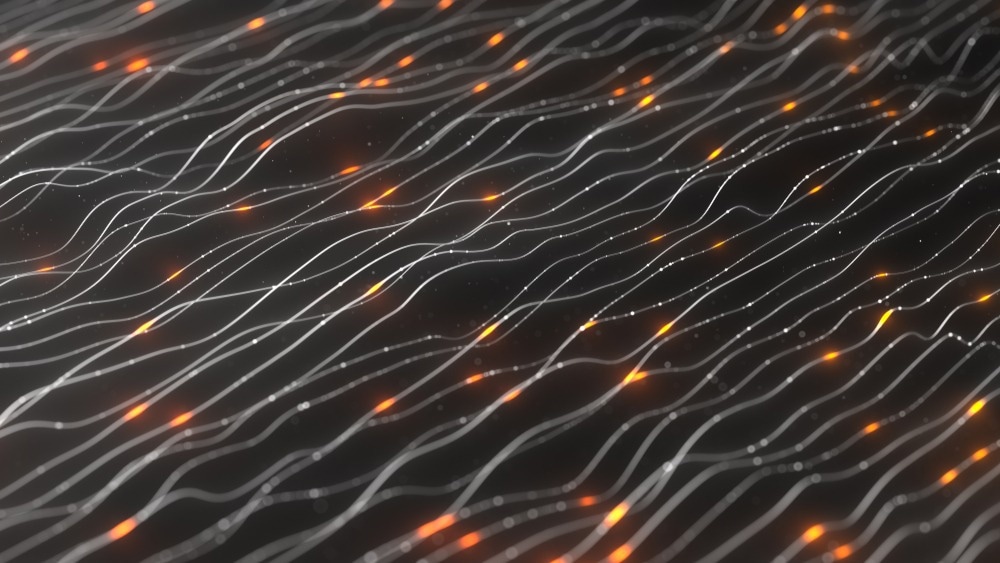
Image Credit: STEVLTH/Shutterstock.com
Recent research explorations have experimented with the concept of applying synthetic dimensions to optics. An approach to optical neural networks using synthetic dimensions in the time domain has been theoretically proposed to use a single resonator network. The arrival times of optical pulses are connected to create a temporal synthetic dimension.
What Are Synthetic Dimensions?
Real dimensions and synthetic dimensions may be combined in 3D space. When matter is investigated in synthetic dimensions, it acts as though it has access to additional spatial dimensions. For instance, 3D systems that are 4D or more advanced. It is sometimes possible to investigate quantum systems more effectively in synthetic dimensions than in actual three-dimensional space.
A photonic structure's apparent geometric dimensionality is frequently used to illustrate its physical properties. Microcavities, waveguides, two-dimensional photonic crystals, and three-dimensional metamaterials are a few examples of zero-, one-, two-, and three-dimensional structures, respectively. Yet, it is actually conceivable to investigate physics in a space with a higher dimensionality than the intuitively seen geometrical dimensionality of these structures using the same photonic structures. The fundamental concept is to set up synthetic dimensions and then combine them with geometric dimensions to create higher-dimensional synthetic space.
Photonic structures have been successfully implemented in optical neural networks (ONN) to computationally mimic the biological neural network of the human brain.
Optical Neural Networks
An artificial neural network with optical components is physically represented by an ONN. Data science could be revolutionized by optical neural networks. The state of data science at the moment focuses a strong emphasis on massive data sets, which are widely accessible thanks to improved computing power and increasingly sophisticated cloud architecture that enable widespread data collection and sharing. Yet, the capabilities of conventional CPUs are beginning to be surpassed in the areas of deep learning and data processing. Deep learning is a subset of machine learning that makes use of artificial neural networks and numerous layers of processing to extract increasingly complex features from input.
The use of optical fields and photonic devices to execute artificial neural network calculations has sparked a broad interest in ONN, spawning a wealth of applications across multiple platforms. Since the total number of layers and the amount of photonic devices in every layer dictate how many photonic devices are required for the majority of the existing ONN systems, further challenging reconfiguration and shrinking are required to implement them efficiently. Finding a different ONN platform that allows for complex and arbitrary functionality is therefore important.
Theoretical Implementation of ONN Using Synthetic Dimensions
Using a single-ring resonator and a synthetic temporal dimension created by the arrival times of optical pulses, a method has been proposed in a new study to develop an optical neural network. In the single resonator network, the optical pulse arrival times are coupled to create a synthetic temporal dimension.
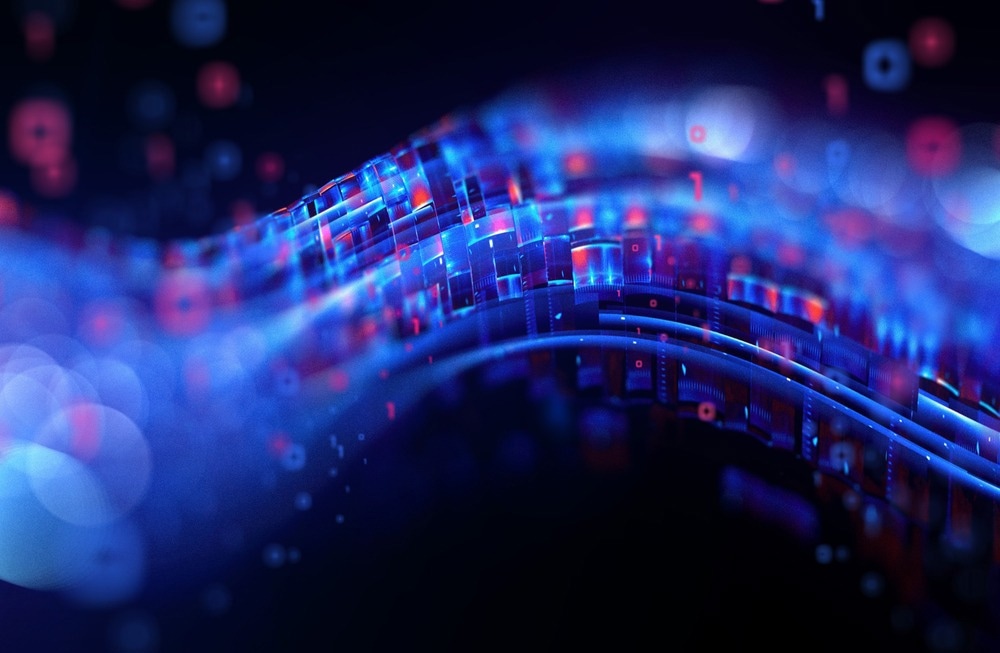
Image Credit: Dmitriy Rybin/Shutterstock.com
Although the arrival time of each pulse denotes the site of every layer, the number of roundtrips is handled as the number of levels. When pulses circulate inside the network, the collection of pulses from each roundtrip serves as the sites for each layer of the optical neural network and can be linearly changed with splitters and delay lines, including phase modulators. By applying modulation phases, which act as the neural network's building block along with a nonlinear optical element for pulses, this linear transformation can be arbitrarily regulated. Such a setup allows for a time-multiplexed lattice with several ONN levels.
Verifying the Functionality of the ONN with Synthetic Dimensions
To intelligently test some of the photonic functionality of the proposed approach, two separate test cases were implemented.
Examples of handwritten digit identification and optical pulse train distribution classification tasks were used to test the functioning of the proposed ONN for deep learning. This computational proof-of-concept study investigates the novel idea of developing a photonics-based machine learning mechanism in one ring cavity network using synthetic dimensions, which enables flexibility and ease of reconfiguration with complicated functionality to accomplish desired optical tasks. The accuracy of our ONN architecture is demonstrated by the prediction errors.
Future Outlook
In conclusion, a novel technique for deep learning utilizing an optical network that is easily reconfigurable is demonstrated. This enables the use of the idea of temporal synthetic dimension to accomplish deep learning using photonic tools in a single-ring resonator. This solution points to a very promising method for carrying out the on-chip optical computation with further downsizing. It demonstrates flexibility and ease of reconfiguration with a large potential for complicated functionality in completing any needed optical tasks.
More from AZoOptics: What Are Semiconductor Lasers and How Are They Used?
References and Further Reading
Sivarajah, I. (11 March 2022) What are Synthetic Dimensions? [Online] AZoQuantum.com. Available at: https://www.azoquantum.com/Article.aspx?ArticleID=303
Luqi Yuan, Qian Lin, Meng Xiao, and Shanhui Fan, "Synthetic dimension in photonics," Optica 5, 1396-1405 (2018)
B. Peng, S. Yan, D. Cheng, D. Yu, Z. Liu, V. V. Yakovlev, X. Chen, and L. Yuan, "Theoretical Proposal of Optical Neural Network Architecture with Temporal Synthetic Dimension," in Conference on Lasers and Electro-Optics, Technical Digest Series (Optica Publishing Group, 2022), paper FTh1A.1
Bo Peng, Shuo Yan, Dali Cheng et al 2023 Chin. Phys. Lett. 40 034201
Disclaimer: The views expressed here are those of the author expressed in their private capacity and do not necessarily represent the views of AZoM.com Limited T/A AZoNetwork the owner and operator of this website. This disclaimer forms part of the Terms and conditions of use of this website.