Histology is the microscopic study of cells and tissues and plays a central role in disease diagnosis. Through the identification of characteristic features in tissue structures, it is possible to diagnose cancers and many other diseases by comparing changes in the cellular structure between healthy and diseased tissues.
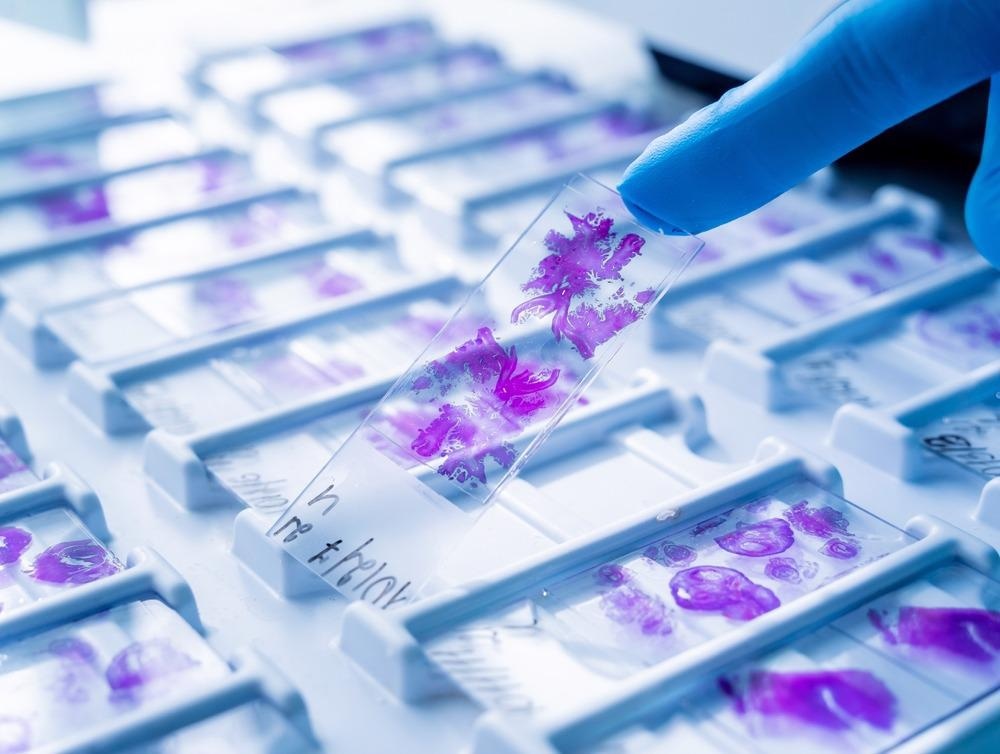
Image Credit: Roman Zaiets/Shutterstock.com
Histology images go beyond simply diagnosing the presence or absence of disease. While a large amount of information in a histology image can be complex to interpret, this information can also be used to grade the severity of tumors.1 Histology is one way of investigating the mechanisms of drug action in the body and whether pharmaceuticals are interacting with the desired target.2
The Role of Microscopes
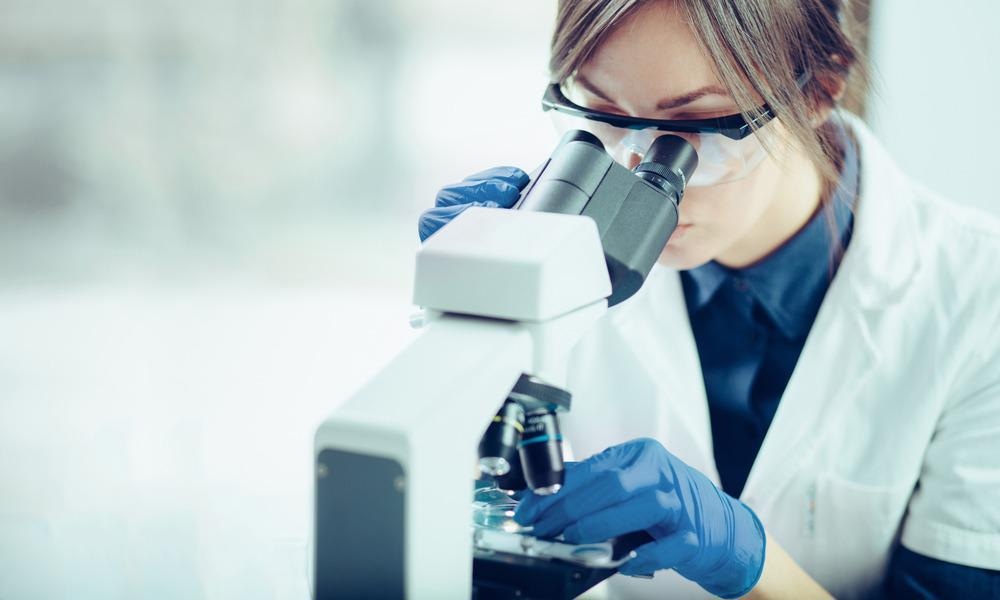
Image Credit: Likoper/Shutterstock.com
As individual cellular structures are too small to be seen by the human eye, microscopy techniques have played a key role in the development of histological techniques.
The original origins of histology lie in the development of optical microscopy techniques that made it possible to visualize cellular structures for the first time.3
Closely related to these developments have been advancements in the use of staining methods. Staining in histology uses chemicals to visualize certain parts of the tissue structure. For example, eosin is a commonly used dye that colors the nucleus of the cell a pinkish shade to make it easier to identify.
As advances in optics have made it possible to perform microscopy with a wider range of imaging techniques and improved spatial resolution, many of these techniques have been adopted as a routine part of histological investigations.
Even techniques such as electron microscopy are now used, and variations on optical microscopies, such as polarized light microscopy, have proved another complementary method to improve the diagnostic accuracy of histology.4
Latest Developments in Histology
Perhaps one of the most significant recent changes in histology work is the use of computer-aided diagnosis. Examining biopsy material with a microscope is an inherently time-consuming process as samples need to be prepared on microscope slides, potentially stained and then the image recorded on the microscope.
The final step of making a diagnosis requires a highly trained individual to examine the image and comment on a potential diagnosis.
Early diagnosis is key to improving the prognosis of many diseases as well as reducing treatment costs6, and for some fast-acting diseases, fast laboratory turn-around times for samples are essential.
With a computer-aided diagnosis, artificial intelligence could potentially replace the role of the highly skilled individual who is required for the interpretation of the image. Improvements in deep learning and automated image recognition mean there is now widely available software that can automatically process histology images and provide a diagnosis.7
Across nearly all areas of medicine, it is known that the clinical’s skill level when assessing an image impacts the probability of a diagnosis. Therefore, part of the appeal of computer-aided diagnosis would be to perform at an equivalent ‘highly skilled’ level without being prone to human error or lapses in concentration.
Trials on computer-aided diagnosis have shown promising results; it was already being used in nearly three-quarters of mammograms in 2010.8 To some extent, computer-aided diagnosis has been used for many years in medicine, but this has been mostly in a support role to a medical expert.
Given the risks associated with missed cancers and false positives, better tools for the interpretation of histology microscopy images are crucial. The volume of data produced, particularly from high-resolution microscopy images, is still problematic to deal with for completely automated analysis and it can be challenging to use for patients with complex disease histories where a complete diagnosis may require the involvement of additional data.
Future developments in this area will need to tackle the issue of dealing with a large volume of complex, unstructured data and to find ways of improving diagnostic accuracy by combining the results of multiple diagnostics tests.
Field Histology
Histology workflows have traditionally involved a sample being taken from a patient and into the laboratory for processing and imaging. However, improvements in point of care devices are making field histology, or histopathology, a possibility.10
Point of care microscopy means using a portable device that can image the cellular information directly from the patient. These are typically non-invasive methods and a number of microscopy techniques can now be used in this way.
While the image quality of many microscopy techniques still benefits from traditional biopsies and sample preparation, there are now ongoing developments in slide-free microscopy methods, all of which are important developments in moving to true in vivo, point of care measurements.
References and Further Reading
- He, L., Long, L. R., Anatani, S., & Thoma, G. R. (2012). Histology image analysis for carcinoma detection and grading. Compt. Methods Programs Biomed., 107(3), 538–556. https://doi.org/10.1016/j.cmpb.2011.12.007
- Judge, A. D., Robbins, M., Tavakoli, I., Levi, J., Hu, L., Fronda, A., Ambegia, E., McClintock, K., & MacLachlan, I. (2009). Confirming the RNAi-mediated mechanism of action of siRNA-based cancer therapeutics in mice. Journal of Clinical Investigation, 119(3), 661–673. https://doi.org/10.1172/JCI37515
- Hussein, I. H., & Raad, M. (2015). Once Upon a Microscopic Slide: The Story of Histology. Journal of Cytology & Histology, 06(06). https://doi.org/10.4172/2157-7099.1000377
- Musumeci, G. (2014). Past, present and future: overview on histology and histopathology. Journal of Histology and Histopathology, 1(1), 5. https://doi.org/10.7243/2055-091x-1-5
- Rivenson, Y., de Haan, K., Wallace, W. D., & Ozcan, A. (2020). Emerging Advances to Transform Histopathology Using Virtual Staining. BME Frontiers, 2020, 1–11. https://doi.org/10.34133/2020/9647163
- Fass, L. (2008). Imaging and cancer : A review. Molecular Oncology, 2, 115–152. https://doi.org/10.1016/j.molonc.2008.04.001
- Lee, S., Amgad, M., Chittajallu, D. R., McCormick, M., Pollack, B. P., Elfandy, H., Hussein, H., Gutman, D. A., & Cooper, L. A. D. (2020). HistomicsML2.0: Fast interactive machine learning for whole slide imaging data. ArXiv. https://doi.org/10.48550/arXiv.2001.11547
- Yanase, J., & Triantaphyllou, E. (2019). A systematic survey of computer-aided diagnosis in medicine: Past and present developments. Expert Systems with Applications, 138, 112821. https://doi.org/10.1016/j.eswa.2019.112821
- Rao, V. M., Levin, D. C., Parker, L., Cavanaugh, B., Frangos, A. J., & Sunshine, J. H. (2010). How widely is computer-aided detection used in screening and diagnostic mammography?. Journal of the American College of Radiology, 7(10), 802-805. https://doi.org/10.1016/j.jacr.2010.05.019
- Calhoun, K., Lin, A., Bryant-Greenwood, P., Lum, C., Johnson, D., & Namiki, T. (2011). Field Histology. Arch Pathol Lab Med, 135, 207. https://doi.org/10.5858/135.2.207
- Jin, L., Tang, Y., Wu, Y., Coole, J. B., Tan, M. T., Zhao, X., Badaoui, H., Robinson, J. T., Williams, M. D., Gillenwater, A. M., Richards-Kortum, R. R., & Veeraraghavan, A. (2020). Deep learning extended depth-of-field microscope for fast and slide-free histology. Proceedings of the National Academy of Sciences of the United States of America, 117(52), 33051–33060. https://doi.org/10.1073/PNAS.2013571117
Disclaimer: The views expressed here are those of the author expressed in their private capacity and do not necessarily represent the views of AZoM.com Limited T/A AZoNetwork the owner and operator of this website. This disclaimer forms part of the Terms and conditions of use of this website.