Confocal microscopes rely on imaging a focused beam of light into a sample. A key advantage of this type of microscopy is its ability to record images without the presence of a fluorescence background.1 This means confocal microscopy can be used for thicker samples and is a label-free technique that reduces sample preparation time.
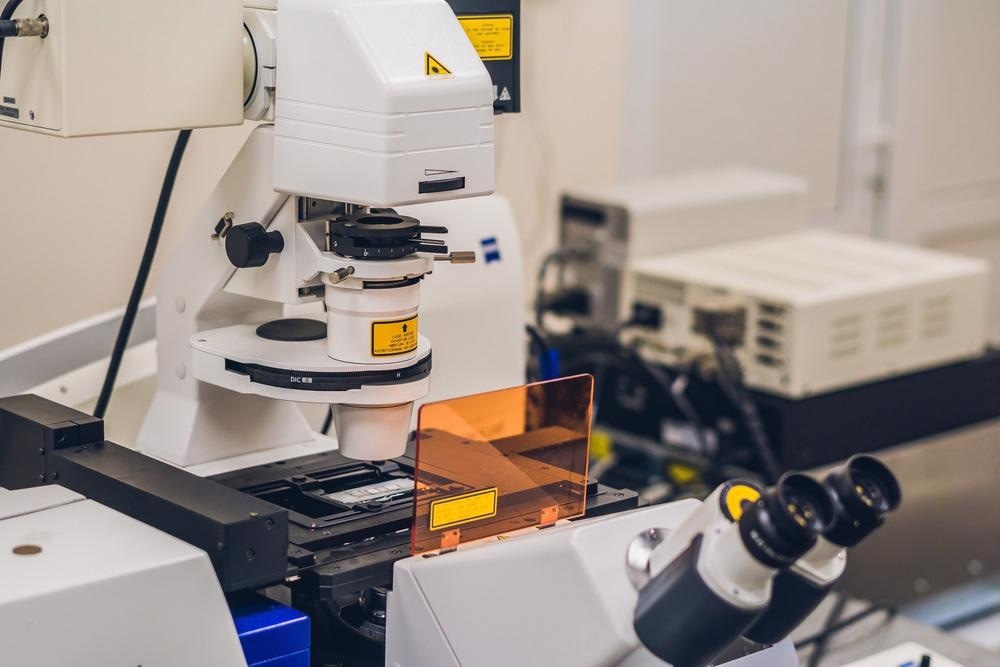
Image Credit: Elizaveta Galitckaia/Shutterstock.com
Confocal microscopy is relatively inexpensive. However, the spatial resolution achieved with the technique has been diffraction-limited – meaning structures smaller than 250-300 nm cannot be resolved in confocal microscopes using visible light sources. This requires the use of super-resolution methods, which are more commonly implemented for fluorescence-based methods.
Although it is now possible to manufacture lenses and optical components to routinely achieve diffraction-limited images, confocal microscopy instrumentation and technique development is still a highly active field.2 Recent developments include the use of lasers as the microscope light source and scanning approaches to image collection. This can either be scanning a sample through translation in the XY plane to construct images of larger sample areas or in the z plane to construct multidimensional images of the sample through different layers.2
While scanning confocal microscopy can be very information-rich and enables full three-dimensional reconstructions of samples, scan times can be very slow. Some sample types also have issues with photobleaching with prolonged irradiation, particularly when more intense light sources such as lasers are used.
A Multiview Platform
Researchers at the Marine Biology Laboratory have used artificial intelligence to improve confocal microscopy, combining different microscopy techniques to improve the imaging platform.3 They have termed this ‘Multiview confocal super-resolution microscopy’.
The microscope platform uses sharp line illumination on the sample and then simultaneously collects the confocal images. It uses fluorescence imaging in epi-mode and for volumes. This requires the use of three objectives to perform essentially three different imaging experiments simultaneously.
By using joint image deconvolution on all of the datasets, the team achieved a lateral resolution of 235 nm and an axial resolution of 381 nm. This is in comparison to a lateral resolution of 452 nm and axial resolutions of 1560 nm using just individual views on the sample. This is a near three-fold improvement in the overall spatial resolution of the image.
To include truly sub-diffraction limited data collection, the team used the diffraction-limited line structure in the microscopy to perform structure illumination microscopy. Super-resolution microscopy images are typically acquired using ‘blinking’ fluorophores that switch on and off in subsequent images. The structure can then be reconstructed using the data from multiple illumination events.
Here, the team isolated any additional fluorescence emission in the region of the line focus and then performed structured illumination microscopy along all three dimensions of the sample. Another advantage as well as the improved resolution is that these experiments could be used for thicker, densely labeled samples as well.
Scanning Efficiency
As sharp line illumination is used for the samples, only a small section of the sample can be imaged at a time. Therefore, the substrate must be translated to construct a scan of the full sample area. With very high-resolution imaging, it is challenging to develop motors with sufficiently fine step sizes and reproducibility to avoid blurring the image resolution.
To achieve this, the team used fiber-coupled microelectromechanical systems (MEMS) in all three views of the sample to achieve scanning areas of 175 µm in each dimension.
A key part of achieving the performance and more efficient scan times with this multi-view microscope platform was the use of deep learning methods.
Super-resolution microscopy is usually reliant on some type of image reconstruction to solve the original structure being imaged. However, for this system, deep learning was also used to help reduce imaging times and avoid issues with motion blur and photobleaching of the sample.
Deep Learning
Using deep learning methods was to better resolve certain features and reduce scan times. As three-dimensional imaging and super-resolution methods typically require more images than conventional microscopy, the team used a residual channel attention network to predict one-dimensional super-resolved images from diffraction-limited images.
The team randomly oriented the images in the training sets and, once the model was ready, applied it to the naturally randomly oriented biological samples. This enhanced the isotropic two-dimensional resolution in comparison to what could be achieved with a single confocal image.
Further enhancements of the platform could be through the use of near-infrared light sources and different lenses to achieve higher spatial resolutions. However, this is an important development in combining different microscopic techniques in a single platform and in exploiting automated algorithms to use the large datasets from such experiments to improve imaging outcomes.
References and Further Reading
- Croix, C. M. S., Shand, S. H., & Watkins, S. C. (2005). Confocal microscopy: comparisons, applications, and problems. BioTechniques, 39, S2–S5. https://doi.org/10.2144/000112089
- Reilly, W. M., & Obara, C. J. (2021). Advances in Confocal Microscopy and Selected Applications. Confocal Microscopy: methods and Protocols (Vol. 2304, pp. 1–35). https://link.springer.com/protocol/10.1007%2F978-1-0716-1402-0_1
- Wu, Y., Han, X., Su, Y., Glidewell, M., Daniels, J. S., Liu, J., Sengupta, T., Rey-suarez, I., Fischer, R., Patel, A., Combs, C., Sun, J., Wu, X., Christensen, R., Smith, C., Bao, L., Sun, Y., Duncan, L. H., Chen, J., … Shroff, H. (2021). Multiview confocal super-resolution microscopy. Nature, 600, 279. https://doi.org/10.1038/s41586-021-04110-0
Disclaimer: The views expressed here are those of the author expressed in their private capacity and do not necessarily represent the views of AZoM.com Limited T/A AZoNetwork the owner and operator of this website. This disclaimer forms part of the Terms and conditions of use of this website.