Computing based on photons offers a faster and more energy-efficient method to handle the ever-increasing demand for maintaining and manipulating data. The Envise platform, developed by Lightmatter, is positioned to disrupt the photonics computing industry with specialized functionalities to positively impact many sectors.
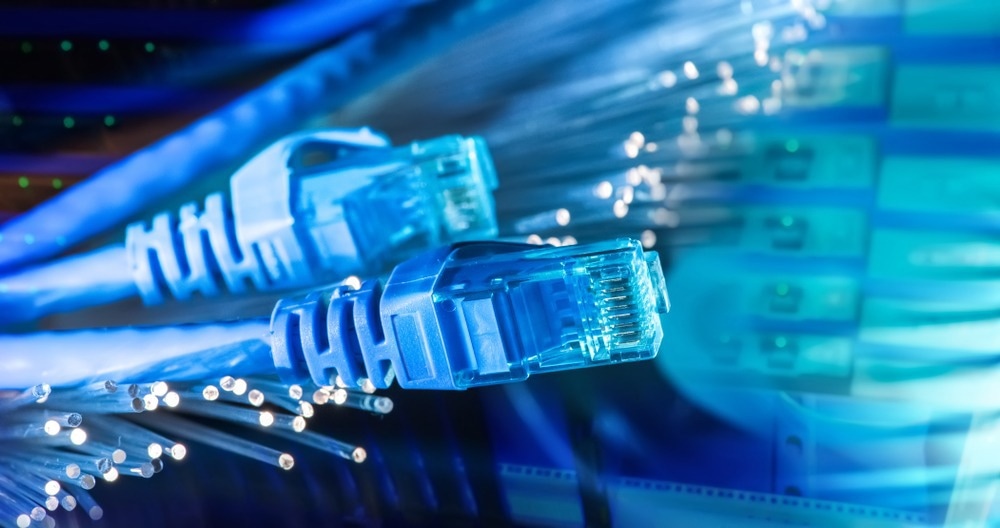
Image Credit: asharkyu/Shutterstock.com
Since the 1940s, when transitioning from analog mechanical devices to digital electronics, computers have been able to move and manipulate electrons in silicon chips, vacuum tubes, and transistors. A new strategy emerged in the 21st Century to address the need for processing ever more data at ever-increasing speeds to enable artificial intelligence (AI) applications using photons for faster and more effective computation.
Although the fundamental concept of optical computing has been experimented with for a while, photonic computing, as it is described now, has been rapidly developed into usable technology. Optical/photonic computing is defined as using photons to compute a particular function or mathematical operation.
Electronic and Photonic Operations
Because photons behave differently than electrons, the early attempts at optical computer technology about 50 years ago proved to be less successful.
Since transistors are nonlinear, they are easier to implement in logic operations. Transistors may flip on and off due to nonlinearity, which enables them to be transformed into logic gates. With electronics, this switching is simple to carry out. Digital computers are particularly adept at using electron-based transistors to control the flow of software instructions.
However, unlike electrons, because photons obey the linear Maxwell's equations, optical devices have proportional inputs and outputs.. It was difficult to construct logic operations with optics since linking together many logic operations depended on the careful control of optical signals. With the technology available at that time, the signals were easily lost with mounting data.
Photonic computing is presenting distinctive opportunities as the computing universe pushes progressively deeper toward AI solutions for an expanding spectrum of applications. The massively parallel processing required for machine learning (ML), artificial intelligence (AI), and deep learning neural network computations are conducive to integrated photonics, which integrates photonic devices on a microchip. Integrated photonics offers much higher computing speed and efficiency while using much less power than electrons.
Envise
Envise, a photonic chip created by Lightmatter, was developed exclusively for artificial intelligence. It is used to run neural networks where calculations like adding and multiplying numbers using linear algebra is carried out frequently.
These additions and multiplications are made possible by integrated photonic components. Linear algebra, a very general mathematical tool, lies at the core of deep learning algorithms and is used to model a wide range of real-world occurrences, from space exploration to financial transactions. These rely on the matrix multiplication operation in linear algebra, which fits the analog linearity of photonics well.
When Google revealed that it was creating a deep learning processor dubbed the tensor processing unit (TPU), the market for AI processor chips was sparked. TPU has a matrix processor array with 256x256 multiply-accumulate units which facilitated the speed up of many of the fundamental computations involved in deep learning. Envise is comparable to Google's TPU work. The electronic core in TPU has been swapped out for a photonic one in Envise. The photonic chip can run much quicker and is also much more energy efficient.
A Mach-Zehnder interferometer (MZI), designed to split and recombine collimated laser beams, is a crucial component of Lightmatter's Envise chip and similar integrated photonics. The matrix multiplication at the heart of the majority deep-learning AI algorithms is carried out by large arrays of MZIs. A program can be executed more quickly and with substantially less energy by utilizing Envise.
With only 3kW of power usage, the Envise 4S has 16 Envise Chips in a 4-U server configuration. A rack-scale Envise inference system that uses 4S as a building block can operate the largest neural networks created to date at unheard-of performance.
Applications
Envise photonics computing has been targeted by many industrial sectors. Some examples are:
- Autonomous driving in the auto industry.
- Enabling predictive and preventive maintenance at manufacturing plants.
- Design of control and vision for robotics.
- Recommendation of a product in e-commerce and advertising.
- Pharmacy, pathology, and cancer detection in the health sector.
- Digital signal analysis and algorithms for signal processing.
- Translation of languages and Text-to-Speech development in language processing.
Limitations and Outlook
There are certain intrinsic limitations in photonic computing that still need further development. Due to the analog rather than digital nature of the calculations carried out by photonic chips like Envise, they may not be as exact as traditional transistors, and system noise may also be an issue. MZIs are an example of a photonic device that is typically larger and cannot yet be crammed onto a chip as tightly as more conventional electronic components.
Even though designers are making great efforts to get around these challenges, it's unlikely that all computers will switch over to photonics chips very soon. AI systems constantly seek out more computing power, hence photonics computing technology offers a way around Moore's law's unavoidable technical limitations.
More from AZoOptics: What Can We Discover with a 3-in-1 Microscope?
References and Further Reading
Envise- Lightmatter. [Online] Lightmatter.CO Available at:
https://lightmatter.co/products/envise/
Wolverton, M. (01 March 2022) Let there be smart light: Photonic computing to power AI and more. [Online] SPIE - The International Society of Optics and Photonics. Available at: https://spie.org/news/photonics-focus/marapr-2022/harnessing-light-for-photonic-computing?SSO=1
Disclaimer: The views expressed here are those of the author expressed in their private capacity and do not necessarily represent the views of AZoM.com Limited T/A AZoNetwork the owner and operator of this website. This disclaimer forms part of the Terms and conditions of use of this website.