Stroke is a major cause of death worldwide. Early identification remains a key strategy to reducing morbidity and mortality in cases of acute stroke. Now, scientists are using artificial intelligence (AI) to improve imaging techniques so that ischemic and hemorrhage strokes might be identified faster.
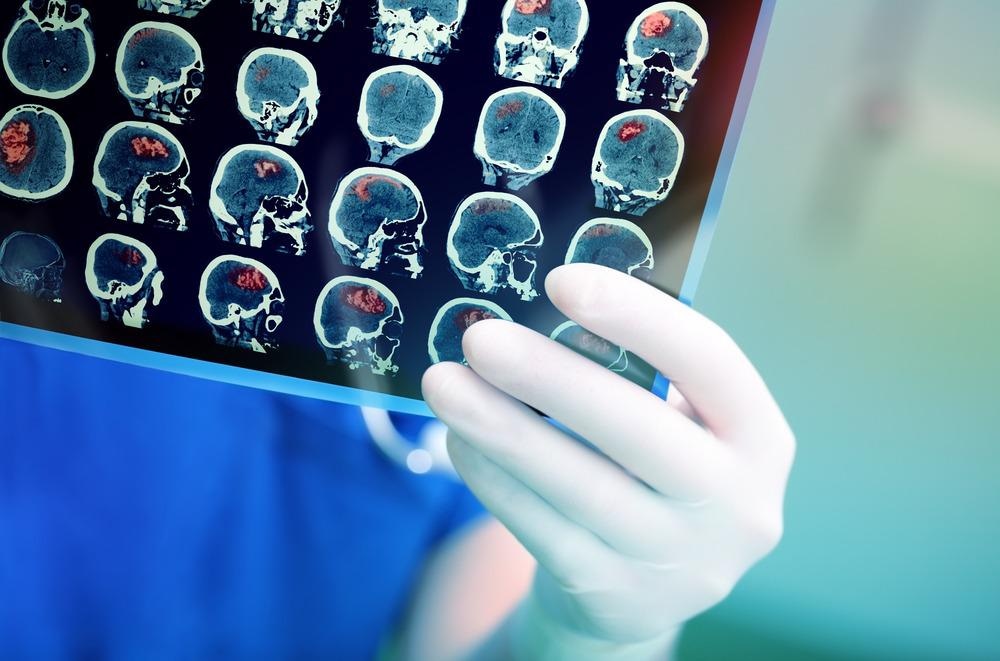
Image Credit: sfam_photo/Shutterstock.com
Recent research has shown that AI can be leveraged in various stages of the stroke treatment paradigm, such as the detection of strokes, Alberta Stroke Program Early CT Score grading, classification, large vessel occlusion detection, prognostication, and segmentation. One line of research that is gaining traction is the development of convolutional neural networks (an AI technique) in performing the image-based tasks outlined above with higher levels of efficiency and accuracy.
Early Diagnosis is Vital for Improving Outcomes of Stroke
Each year, roughly 5.5 million people worldwide die from stroke, making it the second leading cause of death globally. In the US alone, around 800,000 people suffer a stroke each year, with the economic burden of the condition equating to roughly $34 billion annually. Around half of those who suffer stroke go on to develop a chronic disability because of the condition.
Studies have shown that early detection is important for improving survival from stroke and patient outcomes. According to recent research, patients who are treated within 90 minutes of the onset of stroke have increased chances of improvement at 24 hours following stroke onset as well as a favorable three-month outcome in comparison with those who were treated after 90 minutes.
Because of the importance of early diagnosis, scientists have been working on various strategies that can speed up diagnosis and allow patients to get treated earlier. Neuroimaging is currently a vital tool for the characterization, detection, and prognostication of both ischemic and hemorrhagic acute strokes.
Recent studies have shown that neuroimaging methods can be enhanced by AI technology. Scientists have demonstrated that AI has great potential to be used to improve the efficiency and speed of neuroimaging tools. It has been leveraged in various applications, including tools for prediction, quantification, surveillance, and triage. Here, we discuss the full scope of the use of AI together with imaging to detect acute stroke early.
AI Platforms Facilitate Stroke Data Sharing
Machine learning (ML) algorithms require large datasets to perform efficiently. Numerous publicly available imaging datasets for ML in stroke have been established to provide data that is already anonymized, annotated, and post-processed. These datasets test newly developed comparing algorithms designed for diagnosing ischemic stroke.
Over recent years, commercial software platforms have become more widely available. These platforms automatically produce information about various stages of the acute stroke triage pathway and are being integrated into clinical routines, providing healthcare professionals with tools to speed up analysis of ischemic stroke and begin treatment faster.
Evaluating Ischemic Stroke with AI
Due to the narrow window of therapeutic efficacy of thrombolysis, the speed of recognizing patients who are candidates for this therapy is vital. Research has shown that ML algorithms have the potential to speed up the process of ischemic infarction identification from CT or MR images. A recently published study showed how a computer-automated detection (CAD) scheme was successfully developed to identify slight changes in attenuation in patients diagnosed with stroke. The technique proved to enhance the detection of stroke for physicians and resident radiologists working in emergency settings. However, it did not improve the detection accuracy when compared to experienced radiologists due to the high stroke detection rates of this group. The data demonstrates that the use of AI may, in certain applications, be useful in assisting specific healthcare professionals, particularly those who are less experienced.
AI is helping to rapidly identify patients who are candidates for mechanical thrombectomy via enhancing the accuracy of diagnosing large vessel occlusion (LVO). Studies have shown that neural networks can be programmed to detect patients who may have LVO with high levels of sensitivity (97.5%).
ASPECTS grading is a commonly used system for determining the full extent of early ischemic stroke. While it is widely used and considered a useful grading system, it has its limitations in that grading can be challenging and agreement on grading is variable.
Studies have also shown how AI has been successful in determining core infarct volumes on diffusion-weighted images (DWI) via automatic lesion segmentation. Establishing infarct volumes is vital to assisting in patient triage.
Finally, multiple ML algorithms have successfully been implemented to predict clinical outcomes following ischemic stroke. Studies have shown that ML algorithms combined with DWI are more accurate at predicting outcomes than DWI on its own.
This evidence demonstrates that AI has great potential to improve the early detection of stroke and improve patient outcomes. In the coming years, it is likely that we will see further key developments in this field.
References and Further Reading
Marler, J., Tilley, B., Lu, M., Brott, T., Lyden, P., Grotta, J., Broderick, J., Levine, S., Frankel, M., Horowitz, S., Haley, E., Lewandowski, C. and Kwiatkowski, T., 2000. Early stroke treatment associated with better outcome: The NINDS rt-PA Stroke Study. Neurology, 55(11), pp.1649-1655. https://pubmed.ncbi.nlm.nih.gov/11113218/
Mouridsen, K., Thurner, P. and Zaharchuk, G., 2020. Artificial Intelligence Applications in Stroke. Stroke, 51(8), pp.2573-2579. https://www.ahajournals.org/doi/abs/10.1161/STROKEAHA.119.027479
Soun, J., Chow, D., Nagamine, M., Takhtawala, R., Filippi, C., Yu, W. and Chang, P., 2020. Artificial Intelligence and Acute Stroke Imaging. American Journal of Neuroradiology, 42(1), pp.2-11. http://www.ajnr.org/content/42/1/2.abstract
Disclaimer: The views expressed here are those of the author expressed in their private capacity and do not necessarily represent the views of AZoM.com Limited T/A AZoNetwork the owner and operator of this website. This disclaimer forms part of the Terms and conditions of use of this website.