Precise insect identification is the first and most important step in pest management. Insect identification is essential in agriculture to choose the best management approaches.
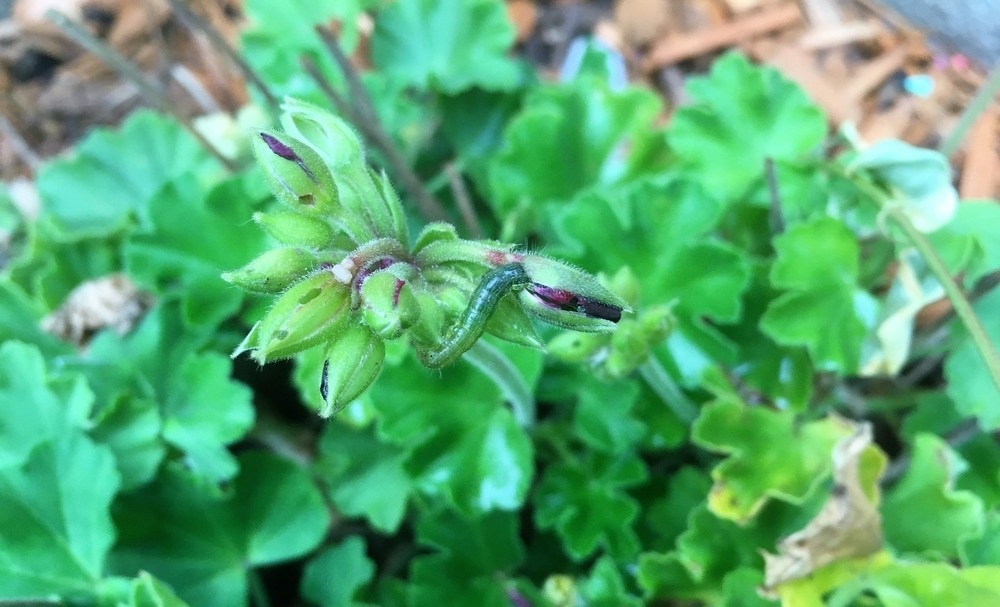
Study: Species Identification of Caterpillar Eggs by Machine Learning Using a Convolutional Neural Network and Massively Parallelized Microscope. Image Credit: jelloyd/Shutterstock.com
The tobacco bollworm (Helicoverpa zea) and budworm (Chloridea virescens) both hatch after five days from the time of oviposition. Helicoverpa zea has developed a tolerance to Bt-transgenic crops, therefore farmers must apply insecticides during the oviposition window. The eggs of Helicoverpa zea are tiny, measuring 0.5 mm in diameter, making it necessary to use a microscope to distinguish between different species.
A recent study published in Agriculture suggests a machine learning approach using a convolutional neural network to classify the different kinds of caterpillars with high accuracy. The researchers created a dataset of approximately 5500 images for training quickly and testing the network owing to the Multi-Camera Array Microscope (MCAMTM), a gigapixel-size parallelized microscope, and an automated image-processing pipeline. In the future, the software could let farmers take an image of eggs on a leaf and instantly identify the species before the eggs hatch.
Damage Caused by Uncontrolled Insects
The tobacco budworm Chloridea virescens (formerly Heliothis virescens) and the bollworm Helicoverpa zea (Lepidoptera: Noctuidae) are significant agricultural pests. When uncontrolled, they cause most pest damage to cotton in the Southeast US.
The larvae consume various agricultural goods, such as cotton, corn, tobacco, soybeans, tomatoes, wheat, and plants in backyard gardens. They are managed in cotton using transgenic plants that express insecticidal Cry proteins from the bacterium Bacillus thuringiensis (Bt), together with the occasional application of foliar chemical insecticides. Unfortunately, Cry protein resistance is spreading and getting worse.
Importance of Insect Egg Identification in Pest Management
Identification is the first stage in managing the US bollworm-tobacco budworm complex in cotton. This enables the adoption of the proper management approach for that particular insect.
The economic threshold for bollworms in cotton was based on the presence of living larvae or damaged plant reproductive tissues before Bt resistance in Helicoverpa zea. After Helicoverpa zea developed Bt resistance, egg-based thresholds were adopted to decide when to spray.
Challenges in Insect Egg Identification
Heliothis virescens and Helicoverpa zea are identical in color, size, and form. The only difference between them is the presence of small cuticular ridges on the surface of Helicoverpa zea. Egg identification requires a high-magnification bright-field microscope and a researcher with extensive experience to determine small cuticular ridges on the egg surface.
When managing common pest populations, this approach is impractical as collecting many samples from fields spread across a wide geographic area is required.
Development of Dataset of Heliothis Virescens and Helicoverpa Zea Using Machine Learning Methodology
Deep learning is being employed more in the biological sciences. Applying convolutional neural networks (CNNs) to digital photographs produce repetitive image classifications. A dataset of images of Heliothis virescens and Helicoverpa zea was developed by Efromson et al.
The researchers employed a Multi-Camera Array Microscope (MCAMTM), and, using the data from this analysis, created a machine learning methodology to distinguish between species quickly.
The subtle and challenging physical changes between two species make this perfect test case to demonstrate the utility and effectiveness of deep learning for identifying insect eggs. Rapid, unbiased, high throughput, repeatable analysis, cheap cost, and a way to enhance the sustainability of insect management are all advantages of this technique.
The Future of Pest Management Using Deep Learning
This research aims to demonstrate the viability of identifying eggs through high-resolution microscopy and machine learning. A computer vision methodology would offer a quickly available, reproducible identification that could otherwise not be available during the little time required for making pest management decisions. For instance, one option could be to take a high-resolution smartphone photo of an insect egg to identify the type of insect.
The researchers discovered that a machine learning method has a 99.3% accuracy rate in classifying images of eggs from two species of caterpillars. The two investigated species represented the worst-case situation in which only minute morphological variations exist between species. In the past, identifying these caterpillar eggs required using anti-body assays from particular species or microscopy to examine minute morphological variations.
The suggested deep learning algorithm only needed a representative high-resolution digital image from the user. It generated an automated, impartial output, in contrast to the labor-intensive, subjective analysis required by the current methods. This is the first account of identifying a species of insects from eggs using deep learning techniques, which has implications for other significant pest management issues.
References
Efromson, J., Lawrie, R., Doman, T. J. J., Bertone, M., Bègue, A., Harfouche, M., Reisig, D., & Roe, R. M. (2022) Species Identification of Caterpillar Eggs by Machine Learning Using a Convolutional Neural Network and Massively Parallelized Microscope. Agriculture, 12(9), 1440. https://www.mdpi.com/2077-0472/12/9/1440/htm
Disclaimer: The views expressed here are those of the author expressed in their private capacity and do not necessarily represent the views of AZoM.com Limited T/A AZoNetwork the owner and operator of this website. This disclaimer forms part of the Terms and conditions of use of this website.