The limited depth-of-field of optical lenses necessitates the use of multi-focus image fusion. Multi-focus image fusion techniques that reduce denoising are crucial as input images are noisy.
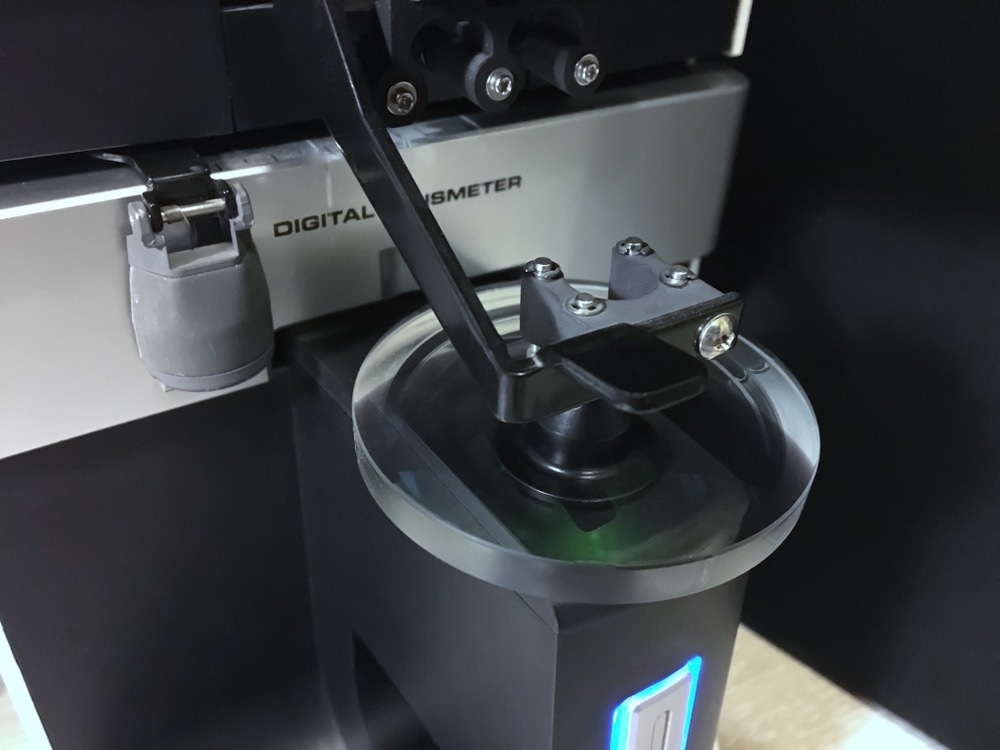
Study: Conditional Random Field-Guided Multi-Focus Image Fusion. Image Credit: taratorkin/Shutterstock.con
A recent study published in the Journal of Imaging presented a conditional random field-guided fusion technique. The Edge Aware Centering method was used to excerpt the high and low frequencies of the images. The Independent Component Analysis transform (ICA) was applied to the high-frequency components. The transform components and low-frequency coefficients were used to build a Conditional Random Field (CRF) model.
The expansion approach effectively solves the CRF model. The predicted labels direct the fusing of the low-frequency components and the transform coefficients. The coefficients of the fused transform are subjected to inverse ICA. Combining the fused high frequency and low frequency is the fused image. By using transform domain coefficient shrinking, CRF-Guided Fusion facilitates image denoising. Both quantitative and qualitative analyses show that CRF-Guided fusion outperforms conventional multi-focus image fusion techniques.
Importance of Multi-Focus Image Fusion
Optical lenses have a limited depth of field. Optical lenses can sharply capture small portions of the image, leaving the rest of the image out of focus or blurry. Multi-focus image fusion methods are crucial for overcoming this constraint. Multiple input images are combined into an image with an enhanced depth-of-field using multi-focus image fusion techniques.
More precisely, the out-of-focus pixels from the input images are discarded, while the well-focused pixels from the input images are kept in the fused image. As a result, the merged image does not introduce artifacts during fusion and has extended depth-of-field.
Limitations of Multi-Focus Image Fusion Techniques
Four types of multi-focus image fusion techniques include deep learning combination, spatial-domain, and transform-domain techniques.
The fused image is calculated using spatial domain algorithms as the average of images. Block-based, pixel-based, and region-based spatial-domain approaches are categorized. When using block-based approaches, the image is divided into fixed-sized blocks, and each chunk's activity level is individually evaluated.
Block-based approaches are likely to have blocking artifacts close to the boundaries of out-of-focus and well-focused pixels since blocks are likely to contain both well-focused and out-of-focus pixels. As a result, the quality of the merged image decreases towards their boundary.
Region-based approaches measure the saliency of the contained pixels by using an entire region of irregular form. Even though region-based approaches offer more flexibility than block-based methods, they can concurrently contain pixels in and out of focus.
Pixel-based techniques have become common recently as a solution to these problems. These techniques estimate activity level at the pixel level. While pixel-based approaches do not cause blocking artifacts and are more accurate close to the border between in-focus and out-of-focus pixels, they are also more likely to result in noisy weight maps and fused images with poorer image quality.
Deep Learning-Based Multi-Focus Image Fusion
Deep learning-based techniques have grown in prominence recently. Deep learning networks anticipate a decision map using a classification-based structure in decision-map-based approaches. Post-processing techniques such as morphological operations are frequently used to improve the decision map. The decision map is utilized to direct the fusion of the input images by choosing the appropriate pixels from the input images.
CNN Fusion, ECNN, and p-CNN are common deep learning decision map-based multi-focus image fusion techniques. Deep learning-based end-to-end networks predict the fused image without needing the decision map's intermediary step. IFCNN and Dense-Fuse are deep learning-based end-to-end networks for multi-focus image fusion.
Conditional Random Field (CRF) Model for Multi-Focus Image Fusion
Bouzos et al. proposed a unique transform domain-based method called CRF-Guided fusion, which uses the Conditional Random Field model to direct the fusing of the transform-domain ICA method. Input images contain noise from a variety of sources. Multi-focus approaches that support fusion and denoising while fusing are crucial.
CRF-Guided Fusion is a multi-focus image fusion-based technique. It supports image denoising resistance and reduces Gaussian noise through the shrinkage coefficient technique. The traditional centering method is replaced with the innovative Edge Aware Centering method (EAC), which reduces artifacts brought on by the centering process.
EAC and the suggested CRF-Guided fusion method work together to produce high-quality fused images that support denoising during fusion while avoiding artifacts for images containing Gaussian noise.
Research Findings
This research presented a brand-new transform domain multi-focus picture fusion approach. The suggested CRF-Guided fusion used CRF minimization, and the labels were used to direct the fusion of the ICA transform coefficients and low frequency, and subsequently, the high frequency. CRF-Guided Fusion provided image denoising during fusion by using coefficient shrinkage.
Modern multi-focus image fusion techniques were outperformed by CRF-Guided fusion, according to quantitative and qualitative evaluation. The transform domain choice and the custom-designed unary and smoothness potential functions for the energy minimization problem were two limitations of the proposed CRF-Guided fusion approach. Future work can utilize CRF-Guided fusion in various transform domains and use deep learning networks to train the unary and smooth potential functions.
Reference
Bouzos, O., Andreadis, I., & Mitianoudis, N. (2022). Conditional Random Field-Guided Multi-Focus Image Fusion. Journal of Imaging, 8(9), 240. https://www.mdpi.com/2313-433X/8/9/240
Disclaimer: The views expressed here are those of the author expressed in their private capacity and do not necessarily represent the views of AZoM.com Limited T/A AZoNetwork the owner and operator of this website. This disclaimer forms part of the Terms and conditions of use of this website.