Pre-proof research from Analytical Chimica Acta suggested a method for non-destructive, fast, and accurate classification and identification of textile fibers based on the one-dimensional convolutional neural network (1D-CNN) model and hyperspectral imaging (HSI) technique.
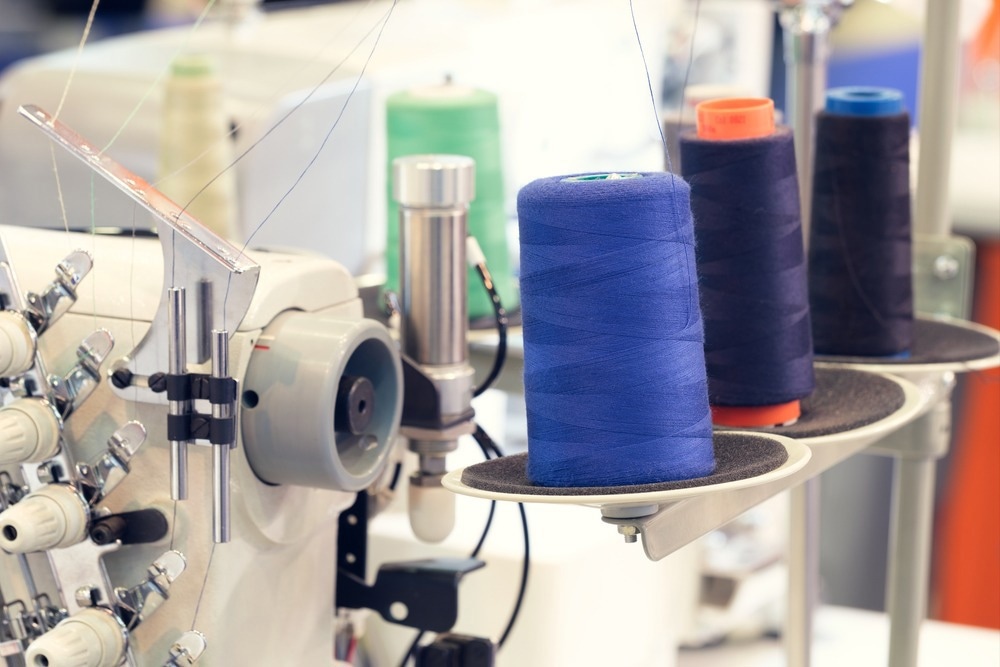
Study: Non-destructive detection and classification of textile fibres based on hyperspectral imaging and 1D-CNN. Image Credit: Matveev Aleksandr/Shutterstock.com
Textile fiber is widely used in everyday life, and its categorization and identification are crucial in textile recycling, public safety and archaeology. However, traditional identification techniques are time-consuming, labor-intensive, and often damage the samples.
This research shows that combining deep learning neural networks with hyperspectral imaging enables a non-destructive method of detecting and classifying textile fibers.
Significance of Classification and Identification of Textile Fibers
Fabrics are made from textile fibers, which may be natural or synthetic. Cotton and wool are natural fibers that comprise plant and animal fibers. Synthetic fiber is a silk thread made of raw fiber material through spinneret holes such as polyethene terephthalate (PET), lyocell, and polypropylene (PP).
Identifying the class fiber is an important part of various industries. Textile fibers may constitute vital evidence at a crime scene and play a critical role in identifying unsolved crimes. Tracing the origin of textile fibers contributes to the resolution of the matter and gives substantial evidence for the lawsuit.
Textile fiber identification in waste fabrics that is both non-destructive and efficient is also essential for repurposing textiles. Therefore, creating a rapid and precise technique for identifying textile fibers is critical.
Traditional Textile Fibers Detection Techniques
The microscopic investigation, visual examination and burning are traditional techniques for detecting textile fibers. With the continual development and advancement of technology, chromatography and spectral approaches are now employed in textile fiber detection techniques.
However, these approaches are time-consuming, have low absorption intensity, poor spectral quality, and excessive baseline noise. As a result, distinguishing textile fibers with these techniques is difficult.
Hyperspectral Imaging and Machine Learning for Textile Fibers Detection
High spectral resolution and multiple bands are distinctive qualities of hyperspectral imaging (HSI). Consequently, HSI has been used extensively in food detection and agriculture, including food categorization, component identification, and pesticide detection.
In image identification, hyperspectral imaging is often accomplished by machine learning techniques. However, typical machine learning approaches have poor computing efficiency and precision for large-volume hyperspectral imaging data. No applicable hyperspectral classification of textile fibers has been conducted to date.
Using the 1D-CNN Model to Detect and Classify Textile Fibers
J. Huang et al. focused on the recognition and classification of textile fiber hyperspectral data by implementing a one-dimensional convolutional neural network and compared it with back propagation neural network (BPNN).
Hyperspectral imaging of 25 different types of commercial textile fibers was denoised and collected by pixel fusion to conduct an accurate, efficient, and reasonable identification investigation of textile fiber evidence.
The hyperspectral data of fiber samples was analyzed using four machine learning classification models: support vector machine (SVM), k-nearest neighbors (KNN), partial least squares-discriminant analysis (PLS-DA), and random forest (RF).
One-dimensional convolutional neural network (1D-CNN) and a back propagation neural network (BPNN) models were prepared and trained to identify and classify the hyperspectral data.
The 1D-CNN model was compared against the four traditional machine learning models and the BPNN model to determine the most accurate and efficient identification model.
Important Findings of the Study
Most textile fibers exhibited little changes, and the forms of the reflectance spectra were quite similar, posing a significant problem and difficulties for intuitive sample categorization. As a result, the hyperspectral data was evaluated using machine learning.
The classification of the textile fiber dataset using standard machine learning (ML) models revealed that the random forest (RF) model performed better than the other three traditional ML models, with an accuracy rate of 91.4%.
The findings revealed that selecting the right neural network was critical. The classification model created by 1D-CNN outperformed BPNN. The accuracy of the 1D-CNN in test sets and training was more than 98%, whereas the BPNN model was less than 88%.
The 1D-CNN model's sensitivity (Se), precision (Pr), F1 score, and specificity (Sp) achieved 98.6%, 98.7%, 98.6%, and 99.9%, respectively. These values were much higher than those of the four conventional machine learning models.
The findings demonstrated that the 1D-CNN model was most stable and efficient at categorizing hyperspectral textile fiber data.
Future Developments
The next step in this research would be to gather more detailed images of textile fiber samples and expand the database. In addition, the neural network model structure and algorithm must be further refined, and the network depth can be suitably raised to boost the precision and accuracy of the detection of samples.
Reference
J. Huang, H. He, R. Lv, G. Zhang, Z. Zhou, & X. Wang. (2022). Non-destructive detection and classification of textile fibres based on hyperspectral imaging and 1D-CNN, Analytica Chimica Acta. https://www.sciencedirect.com/science/article/abs/pii/S0003267022008091
Disclaimer: The views expressed here are those of the author expressed in their private capacity and do not necessarily represent the views of AZoM.com Limited T/A AZoNetwork the owner and operator of this website. This disclaimer forms part of the Terms and conditions of use of this website.