Monitoring areas susceptible to natural disasters has become a global priority. Effective methods and pertinent land cover data is required to decrease disaster risk. In a study published in the journal Sensors, researchers created a high-resolution land cover map of flood-prone rural settlements using multispectral drone data.
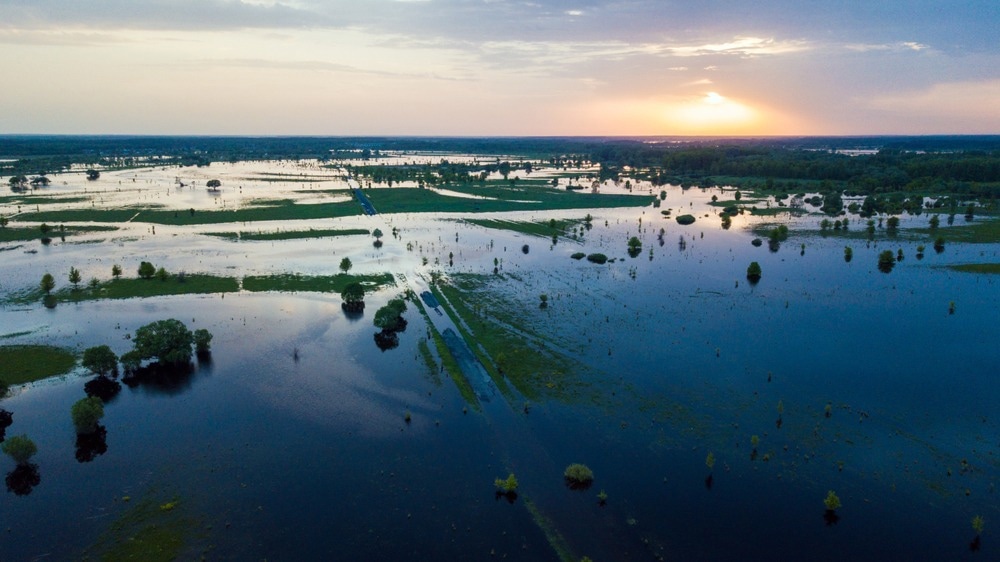
Study: Land Cover Classification from Very High-Resolution UAS Data for Flood Risk Mapping. Image Credit: Vadven/Shutterstock.com
Monitoring of Vulnerable Areas
Extreme floods threaten the safety of a region and can cause substantial damage. Planning against climate change hazards is essential to protecting people, communities, and nations, as well as their livelihoods and health, economic assets, cultural heritage, and ecosystems.
Monitoring the world's most susceptible locations to natural disasters has become a global priority.
Sendai Framework for Disaster Risk Reduction
The Sendai Framework is an international agreement that aims to reduce disaster risk and losses to lives, livelihoods, health, and economic, cultural, physical, social, and environmental assets of businesses, individuals, communities, and countries.
Insufficient resistance to natural disasters can weaken or stop the progress of sustainable development goals (SDGs).
Significance of Land Cover Maps
Understanding the relationship between land cover (LC) and geophysical hazards is essential. The LC maps offer critical information for evaluating and developing flood risk management strategies. Therefore, land cover affects the flood itself in one way or another.
Land cover mapping provides data on flood-prone assets, including agricultural land, infrastructure, and human settlements.
Urban areas, soil, agricultural regions, and plants have varying permeability. The domination of one over the other, or an imbalance in their distribution, significantly affects flood behavior.
Infrequent use of UAS data for LC is due to complex automated categorization owing to significant radiometric heterogeneity and poor spectral resolution.
Object-oriented classification approaches effectively identify the high-resolution land cover in images, such as those obtained by unmanned aerial systems (UAS).
Several studies employed different methods and models to classify land cover from very high resolution (VHR) optical data. They differed primarily in training sample size, approach, and input dataset.
Using Multispectral Drone Imagery to Generate a High-Resolution LC Map
Belcore et al. defined high-thematic resolution land cover classes related to flood risk mitigation planning.
Two photogrammetric unmanned aerial systems (UAS) flights were performed with fixed-wing NIR and RGB optical sensors. The LC input dataset was created using the standard structure from motion (SfM) method, yielding a digital surface model (DSM) and two orthomosaics.
The LC system comprised nine classes calculating flood-related potential losses such as residences and production sectors. A random forest (RF) classifier helped produce the LC using object-oriented supervised classification.
Textural and elevation parameters were calculated to address mapping challenges caused by the high spectral uniformity of cover types.
Special consideration was given to the classes' definition to meet flood risk management standards and appropriately identify flood-exposed structures from a geometrical standpoint. The buildings were subjected to geometric validation as part of the segmentation process.
The training-test dataset was manually created.
Important Findings of the Study
The segmentation was completed in 11 hours and consisted of 34,439 items, whereas the classification took around four hours.
The segmentation yielded an F1 score of 0.70 and a median Jaccard index of 0.88. The RF model had an overall accuracy of 0.94 except for rocky concentrated regions and grasslands.
The area-based assessments did not support the segmentation method's tendency to over-segment. The over-segmentation average's median value was 0.32, whereas the under-segmentation index was 0.63.
The demand for more input characteristics was directly related to increased spatial and thematic resolution, and textural information was proven critical in the classification and segmentation processes. The grey level co-occurrence matrix (GLCM) derived measurements significantly impacted the classification and segmentation procedures.
The single pixel of a particular feature of the scene element has a significant amount of spectral variance due to the high spectral diversity of very high-resolution (VHR) imagery. This is the primary reason for the widespread use of object-based image analysis (OBIA) approaches in categorization. However, the segmentation relies heavily on the analyst's expertise.
Although the final classification accuracy is satisfactory for the risk reduction strategy, further study is required to identify shareable and efficient segmentation methodologies.
The final classifications met the demands of the planners and overcame the criticalities associated with the study area's high cover variability and spatial resolution.
The final precision is sufficient for disaster risk planning, allowing for the exact identification of exposed facilities and calculation of probable flood-induced losses in the region.
Reference
Belcore, E., Piras, M., & Pezzoli, A. (2022). Land Cover Classification from Very High-Resolution UAS Data for Flood Risk Mapping. Sensors, 22, 5622. https://www.mdpi.com/1424-8220/22/15/5622
Disclaimer: The views expressed here are those of the author expressed in their private capacity and do not necessarily represent the views of AZoM.com Limited T/A AZoNetwork the owner and operator of this website. This disclaimer forms part of the Terms and conditions of use of this website.