Forensic evidence is often pivotal in reaching verdicts in legal cases. Given the challenges associated with finding eyewitnesses for many crimes and the fact that forensic evidence, in particular DNA evidence, is highly regarded by jurors, forensic methods that can be used to recover genetic information even in trace amounts from samples are very important for the criminal justice system.1
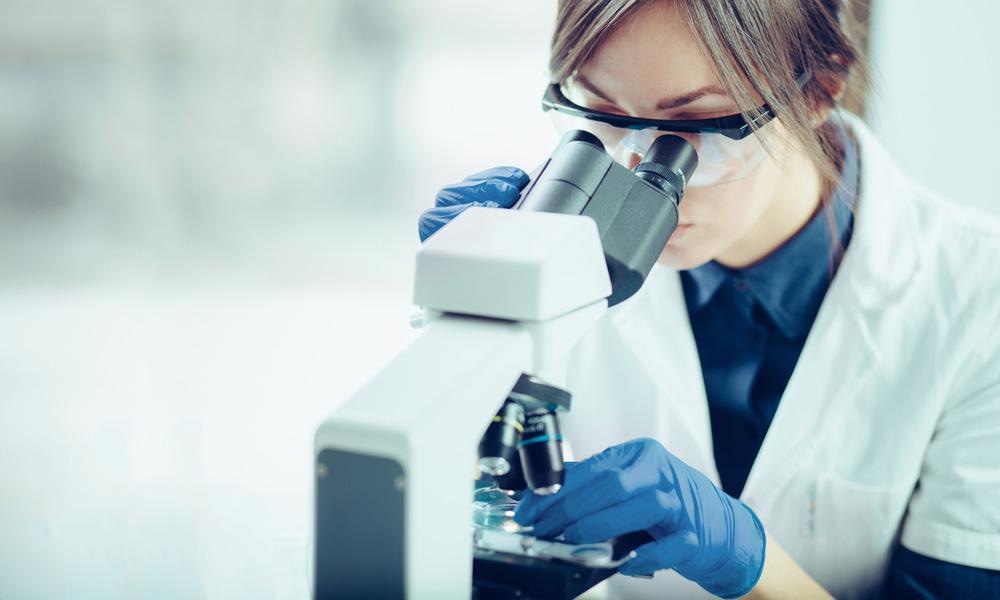
Image Credit: Likoper/Shutterstock.com
Acquiring high-quality evidence from biological fluid samples can be challenging. Often, there will only be a small amount of material to work with and many biological samples will undergo chemical degradation rapidly, particularly if they are exposed to changing weather conditions.
For semen samples, the most common method of identification is the recognition of sperm cells from the sample. This is often performed using staining or fluorescence tagging methods to help visualize the sperm cells under microscopic examination.2 However, for samples from individuals who are azoospermic – meaning there are no sperm cells present in the semen sample due to blockages or vasectomies, this is not an appropriate method of sample identification.
Alternatives include detection of seminal fluid proteins or the use of antigen tests3 but often these do not have good specificity. Extraction of RNA and DNA requires large sample volumes and is a relatively complicated procedure to perform so microscopy is still preferred for its relative simplicity and the speed of the analysis that can be performed.
Automated Microscopy
A new approach that is being tested for feasibility for sperm identification with optical microscopy techniques is the use of deep convolutional neural networks as an image analysis tool.2 The advantage of these automated analysis methods is that they can be combined with an automated recording of microscope images.
Microscopes use focused light to create magnified images of an object. Typically, the tighter the focus, the greater the spatial resolution that can be achieved in the imaging process. However, this also means that a smaller section of the microscope slide can be imaged at a time and so scanning a sample of a given area will take much longer.
As translational stages can be used to move the sample and scan over the area of interest in an automated fashion, these can be combined with automatic image processes to investigate relatively large slides in some amount of detail. Convolutional neural networks are also excellent at ‘classification’ tasks, and in this work, the researchers would classify images into two categories – sperm cells present or sperm cells absent.
One complication with using automated image recognition for cells is that cells can be deformed, obscured by the other cells and material that are present, or can be inconsistent in how they respond to the staining process.
Convolutional Neural Networks
A successful machine learning approach such as using a convolutional neural network relies on the availability of good training datasets of sufficient size that the algorithm can ‘learn’ from a set of known images. This is relatively straightforward for forensics where extensive datasets exist.
For this work, researchers tested the model on a total of 1,942 microscopy images. These were sampled for different areas on the patient as well as from clothing samples. The swabs had been recovered using different collection techniques. The amount of pressure used in the swabbing process and the staining quality impacts the overall quality of the image, so this set was considered to cover a good range of conditions.
For the training sets, the images were rescaled to 345 x 256 and underwent a cropping process to make them 256 x 256 pixels. They could then be augmented to extend the size of the training set through techniques such as image rotation.
Results
For forensic work, any evidence must be examined by two experts. The researchers use the neural network to identify regions where the algorithm identifies sperm cells and flag these to speed up the human analysis of the images. This also made it possible to identify that image characteristics could potentially lead to false identifications.
Overall, the algorithm only showed nine misclassifications of images, with most of these being examples of sperm-positive images that had been classed as negative. Most of these were unusual staining colors, with lots of debris present and a very limited number of cells.
The two network models trialed showed an accuracy of over 90% and the ability of one model type to generate bounding boxes to indicate likely regions sperm cells were present in significantly speeding up human reanalysis of the images. While tests against human experts to evaluate the relative accuracy still need to be performed, this approach forms the basis for using automated microscopy for forensics analysis and helping to streamline and accelerate the sample analysis process.
References and Further Reading
- Lieberman, J. D., Carrell, C. A., Miethe, T. D., & Krauss, D. A. (2008). Gold versus platinum: Do jurors recognize the superiority and limitations of DNA evidence compared to other types of forensic evidence?. Psychology, Public Policy, and Law, 14(1), 27. https://doi.org/10.1037/1076-8971.14.1.27
- Golomingi, R., Haas, C., Dobay, A., Kottner, S., & Ebert, L. (2022). Sperm hunting on optical microscope slides for forensic analysis with deep convolutional networks – a feasibility study. Forensic Science International: Genetics, 56. https://doi.org/10.1016/j.fsigen.2021.102602
- Hochmeister MN, Budowle B, Rudin O, Gehrig C, Borer U, Thali M, Dirnhofer R. (1999) Evaluation of prostate-specific antigen (PSA) membrane test assays for the forensic identification of seminal fluid. J Forensic Sci. Sep;44(5):1057-60. PMID: 10486959. https://seratec.com/docs/Hochmeister_1999.pdf
Disclaimer: The views expressed here are those of the author expressed in their private capacity and do not necessarily represent the views of AZoM.com Limited T/A AZoNetwork the owner and operator of this website. This disclaimer forms part of the Terms and conditions of use of this website.